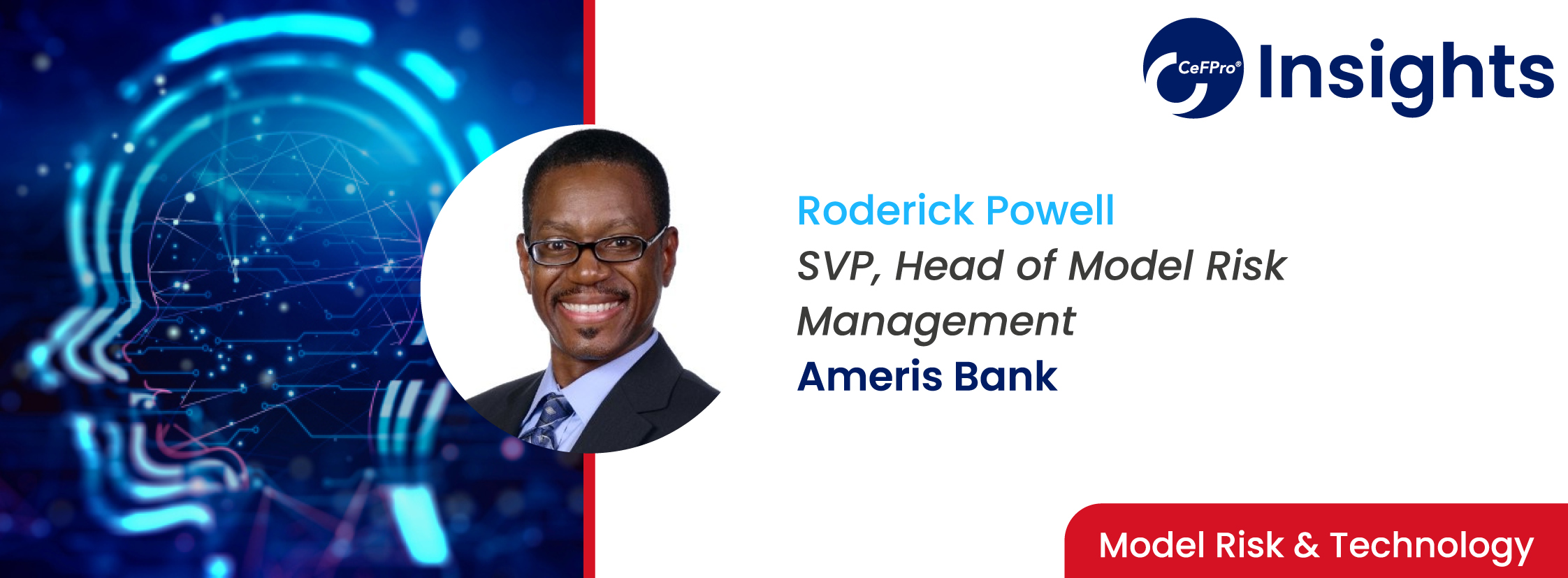
Assessing and Monitoring AI, machine learning, and large language models to avoid bias and toxic results
Roderick Powell, SVP, Head of Model Risk Management, Ameris Bank
Below is an insight into what can be expected from Roderick’s session at Advanced Model Risk USA 2024
The views and opinions expressed in this article are those of the thought leader as an individual, and are not attributed to CeFPro or any particular organization.
How can organizations effectively assess and continuously monitor AI, machine learning, and large language models to prevent and mitigate bias and the generation of toxic results in their applications?
There are various tactics that organizations can use to assess models that are in use for potential or actual bias. There are two tactics to do this that come to mind: 1) Employ bias detection algorithms, and 2) Red-teaming.
There are many open-source bias detection algorithms available in Python. These algorithms and tools are designed to detect and measure bias within AI systems. They can help identify areas where the model’s performance is not equitable across different groups. Red teaming is another tactic that can be used to identify bias. Red teaming involves using humans to stress test models to discover if this leads to biased outcomes. Red teaming is particularly useful for large language models and other generative AI applications. It is also important to conduct periodic audits of AI models to assess their decisions for fairness, accuracy, and potential bias.
Bias can be mitigated on the front end (data), middle (training), and back end (output) of models. On the front end, organizations should ensure that model training data is comprehensive and representative of diverse demographics to minimize the risk of bias. Datasets should be checked for imbalances, oversights, or historical biases. Adversarial training can be used to stress test model results. It can be used to determine how certain inputs can adversely alter model results. In the context of large language models, the key methodology used for mitigating risk during the training process is Reinforcement Learning with Human Feedback or RLHF. This is a way to fine-tune a model to make its results more aligned with positive human values.
Specific human values for a given company can be spelled out in a Constitutional AI document. This document can be used to inform AI model development. It is important to have a diverse team (in terms of ethnicity, gender, etc.) draft the Constitutional AI document. In addition, the team that drafts the guiding document should not just be comprised of technologists, but also people from other domains.
On the back end, model output or decisions should be reviewed by humans. Human-in-the-loop systems can be used to review and override AI decisions that are identified as potentially biased or harmful. In addition, guardrails can be put in place to prevent large language models from generating toxic results.
What strategies can organizations employ to strike a balance between model performance, robustness, and fairness when implementing AI and machine learning models?
Organizations should frame model training as a multi-objective optimization problem, where performance, robustness, and fairness are all optimization targets. Organizations should choose models that are appropriate for the problem being addressed. Model developers should not use model performance as the sole determinant of model suitability, but also consider a model’s fairness. In the banking sector, this is especially relevant for models that approve or deny loans.
How can organizations effectively manage uncertainties associated with data acquisition to ensure that their models remain free from bias?
First, organizations should maintain transparency about where and how data is collected to ensure model developers and owners understand the potential for biases introduced by the collection methods and the contexts. Training data can be collected from varied sources to reflect the diversity of the population for which the model is being developed. Collecting and curating diverse and representative training datasets can mitigate biases. Care should be taken when using certain features or predictors in models. For example, bias can creep into a model indirectly by using features such as zip codes in a model.
A thorough initial data analysis should be done before model development to identify and address potential sources of bias. Then data sources should be continuously monitored for potential biases that may evolve over time as societal norms and populations change.
What strategies should organizations consider when extending their MRM functions to build models that prioritize explainability and thorough bias testing in AI and machine learning applications?
MRM is a second-line risk management function and should be kept separate from a first-line model building function. However, MRM can certainly promote the building of models that are not biased and are transparent. Developers should consider building simpler models if they offer sufficient performance with increased transparency and interpretability. There is not always a direct correlation between model complexity and performance. Model developers should proactively identify and mitigate bias in their models.
MRM should address the model development process in model risk management policies and programs. MRM should also ensure that model developers and owners maintain comprehensive model documentation. In addition, model validation procedures for AI and machine learning models should specifically include an assessment of model transparency and potential bias.
MRM may develop AI and machine learning models to use for their own work-related tasks. In those situations, MRM should not validate their own models but use an independent, third-party validator to avoid a conflict of interest.