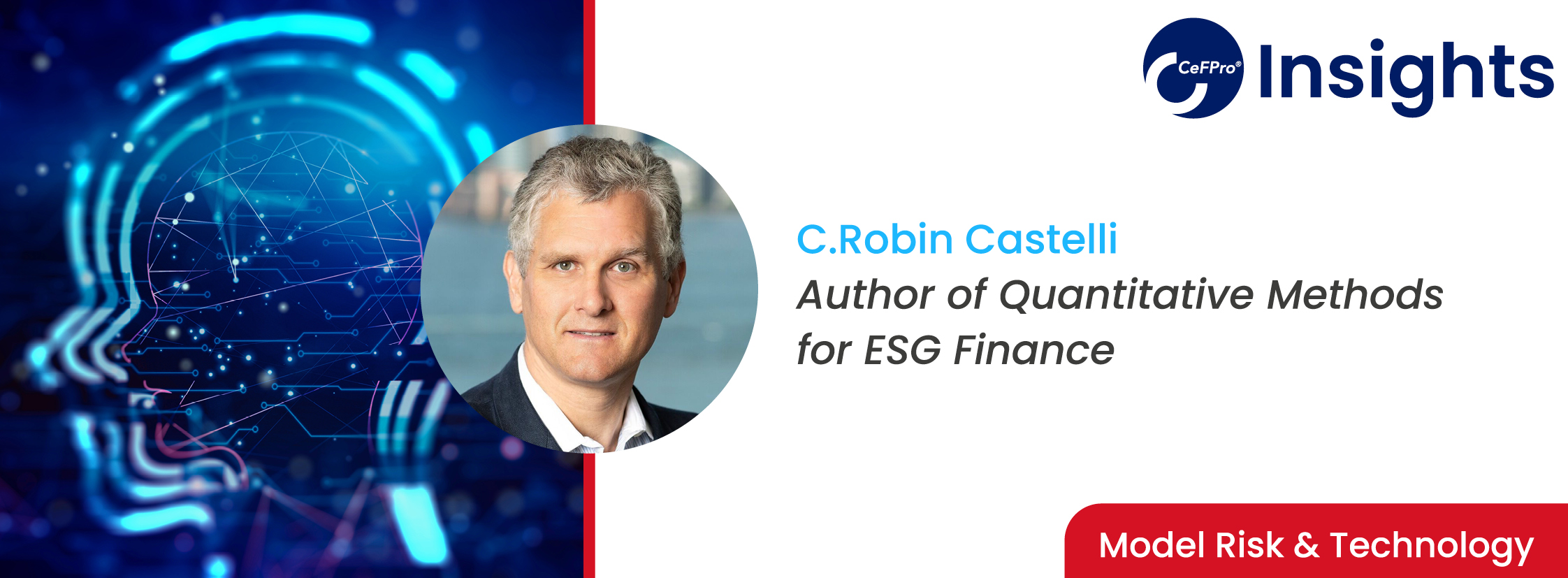
Reviewing the impact of climate risk and incorporating within model risk management
C.Robin Castelli, Author of Quantitative Methods for ESG Finance
Below is an insight into what can be expected from C.Robin’s session at Advanced Model Risk USA
The views and opinions expressed in this article are those of the thought leader as an individual, and are not attributed to CeFPro or any particular organization.
What data can be used to cross-reference climate impact on modeling portfolio?
Climate impacts on a portfolio can be attributed to the transmission channel that drives them, typically as follows:
- Transition risk: The impact comes as the result of societal responses to changes in temperature and precipitation, changes in ecological productivity, and rising sea levels. These societal responses typically take the form of new policies and regulations (limits on emissions, carbon taxes, etc…), development of new technologies, and changes in consumer behavior. The societal response then drives macroeconomic effects such as changes in asset values, increases in costs, and decreases in productivity, and results in impacts on macroeconomic variables such as GDP, fiscal revenue, interest rates, exchange rates, and many others. This macroeconomic effect then cascades on the clients of financial institutions by affecting their CapEx, balance sheets, and cost/demand/profitability structure. Finally, these effects at the client level impact the financial institutions at multiple levels, such as the cost of credit (CRE, RRE, C&I, and Counterparty Credit Risk), the risk for bank-owned properties, market risk (due to the repricing of commodities, credit, equities, fixed income, etc…) and the overall effect of net-zero commitments.
- Chronic physical risk: The transmission channel and climate effects here are the same as for transition risk, and the societal response driving the impact is the issuing of new Policies and Regulations. The effect of these new policies and regulations (typically energy efficiency mandates, mandates not to build in certain areas, etc…) at the macroeconomic level is seen in sectoral GVA impacts, Impacts on real estate valuations, loss of household wealth, and the need for corporate mitigation strategies. These macroeconomic effects hit the clients of financial institutions at the balance sheet level (decreasing value of assets) as well as affecting their cost/demand/profitability structure. The resulting impact on financial institutions is therefore focused on CRE and RRE cost of credit.
- Acute physical risk: In this case, the climate driver is the higher frequency of extreme weather events (heatwaves, coastal flooding, hurricanes, wildfires, cyclones) and their impacts. As in the case of chronic physical risk, the societal response comes in the form of issuing of new policies and regulations. The macroeconomic impact of the societal response takes the form of sectoral GVA impacts and impacts on real estate valuation, which leads to clients of financial institutions being hit by higher insurance costs, damage to their RE holdings, and business disruptions. At the financial institution level, these effects lead to impacts on C&I cost of credit, CRE cost of credit, and RRE cost of credit, as well as a direct impact on bank-owned properties.
- Climate liability risk: This risk does not derive directly from climate effects in the first order but is rather the result of increased awareness of climate change within society. The Societal response is an increase in Climate-linked litigation, which leads, at the level of financial institutions’ clients, to higher liability and compliance costs and increased business disruption. The impact on the financial institutions themselves is in the domains of C&I cost of credit, reputational risk, KYC, legal and compliance costs, and bank business liability risk.
What effect does climate change have on providing loans?
Climate risk is a risk that needs to be properly identified and measured and a risk appetite statement issued. It is a complex and multi-faceted category of risk, with most of the losses being concentrated in the tails of the distribution, where runaway scenarios occur with multiple effects happening concurrently (physical risk into transition risk, concentration risk, climate liability risk, stranded assets, network risks, etc…). In order to have a proper risk management framework in place to address climate risk in a prudent manner, all of these facets need to be considered via the use of new modeling frameworks, such as the regulators have been discussing in recent conferences (ref. Bank of Canada stress test), to allow for the identification and highlighting of these emerging risks. Once the risks and tail events have been identified, the framework for actually integrating these risks into the credit E2E process already exists, and there is no real need for any new development activity (i.e., once we know what the financials for an obligor will look like, we know what the PD/LGD/EAD is and how much credit can be granted, and the driver for the financial condition is not a relevant metric).
How can we effectively model both physical and transition risks associated with climate change within our risk management strategies?
The main challenge is to be able to focus on the climate premium and leverage the existing frameworks for PD/LGD/EAD, as these are based on the financial conditions of the obligors and would be no different for a climate-driven bankruptcy than they would be for a “vanilla” one (with the exception of a few climate-specific overlays that need to be included, such as Stranded Assets considerations). The focus needs to be on the translation of the climate scenarios (effectively the output of the IAMs) into impacts on individual obligors while considering all the concurrent effects that the scenario will have (i.e., physical risk and transition risk happening together, and not run as independent events, plus all the other additional second and third order effects that drive the tail events). While the initial impact (emissions driven for transition risk and direct impact for Physical) are relatively straightforward to model, these are rarely the drivers for significant risk in a portfolio. The true risks, which are not visible if the analysis stops at first-order consequences, come from runaway scenarios and combined effects, which need to be modeled computationally with very large numbers of runs. This requires new modeling techniques and model validation paradigms in order to adapt to the challenge of modeling a transition from a well-known state to one that has never been seen before (and for which no back-testing can be performed).
What strategies can be employed to effectively manage the absence of data when conducting climate stress testing?
The problem of data for climate stress testing is dualistic in nature. There is a lack of data, for many companies, on one side, and additionally, there is unreliable data for many others.
The strategy to deal with these two problems is different, as is to be expected.
For missing data, the current go-to solution is to impute data using sector/geography/industry averages adjusted by company size when it is not possible to find alternative sources for company data (albeit the alternative sources then have issues of reliability).
For reported data, the problem becomes one of checking the accuracy of the data itself, both for errors and deliberately under-reported numbers (this is especially common with emissions, even more so when looking at methane emissions that have been historically hard to track). For these cases, what is typically done is to review the emissions against the industry averages and identify outliers for in-depth review. An additional strategy, especially for methane or emissions in general, is to rely on third-party data providers who can independently provide assessments on corporate emissions, including direct measurement of methane, and compare these results to the company-reported ones to get an idea of the % of under-reporting that an individual company is engaging in (this then allows the model developers to use this scaling factor for modeling purposes).
How does the specific stress caused by climate-related factors impact the path of macroeconomic variables?
The standard climate models that assess the change in climate, given a pathway of GHG emissions, are the GCMs (General Circulation Models), which are purely climatological models. There are also models that assess the impacts and damages of the changes on the economy and on specific sectors. The combination of these two types of models creates a new class of models called Integrated Assessment Models (IAM). The IAMs are what is used to generate the SSP projections both for physical parameters (Temperature, GHG emissions) and macroeconomic variables (GDP and similar). Most IAMs are general equilibrium (optimization) models, but there are also more traditional econometric models in use as well as agent-based models.
Most IAMs are built by combining two modules, an economic module and a climate module, which feed off each other, and are used to maximize a specific utility function.
Typically the modules fall along the following lines:
- Economic module: defines the production function (GDP), including the impact of climate change on GDP (mitigation and adaptation costs, physical damage costs); the quantitative impact is calculated as a function of the average temperature, which is provided by the climate module.
- Climate module: a model of atmospheric and oceanic temperature distribution, including the dynamics of GHG emissions, based on assumptions and scenarios, some of which are exogenous (ab initio parameters) and others deriving from the economic module itself.
Many of these models are open-source and can be run online, using the cloud capabilities of large NGO organizations, but require a significant understanding of the underlying science and economics (some models require GDP as an input, such as GCAM, while others, such as the MIT models, actually produce GDP as output).