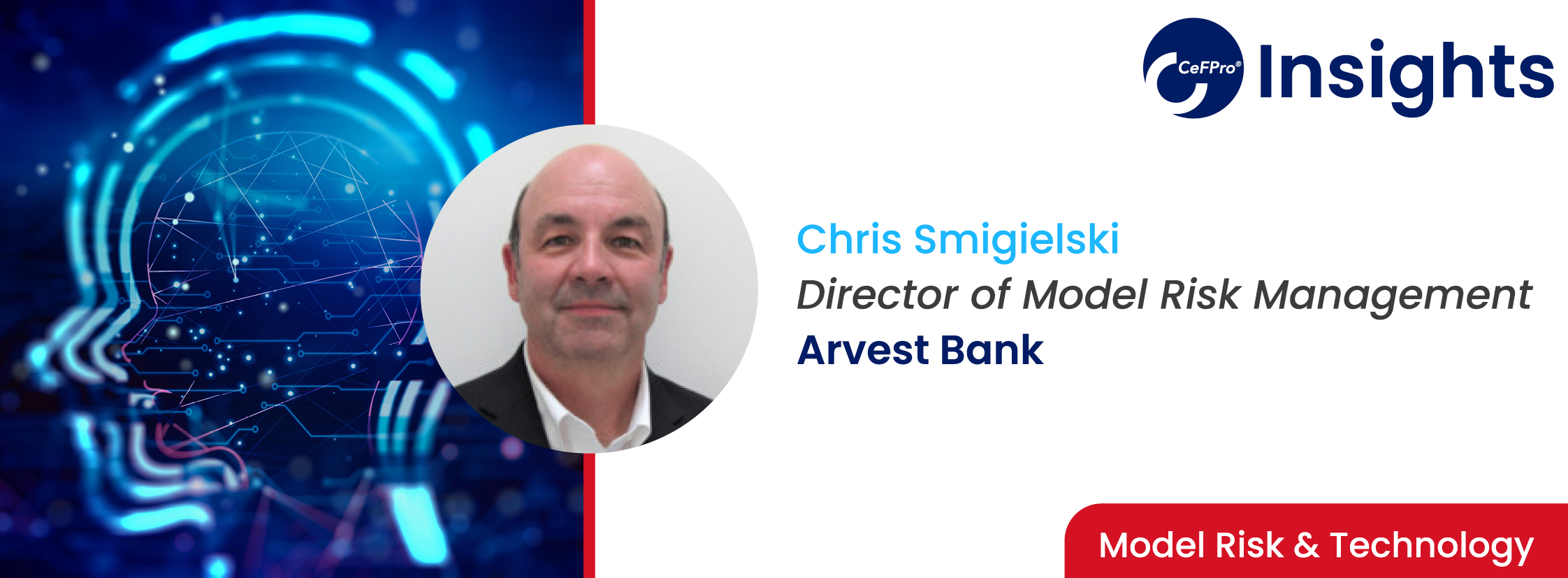
Implementing guardrails to ensure the responsible and ethical use of AI
Chris Smigielski, Director of Model Risk Management, Arvest Bank, NFR Leaders Advisory Board member, CeFPro
Below is an insight into what can be expected from Chris’ session at Risk Americas 2024.
The views and opinions expressed in this article are those of the thought leader as an individual, and are not attributed to CeFPro or any particular organization.
-
How can AI be used to streamline customer communication?
AI offers various tools and techniques to streamline customer communication in banking. Natural Language Processing (NLP) is a cornerstone technology that enables AI systems to understand and respond to customer queries efficiently. By implementing chatbots, virtual assistants, and automated email responses powered by NLP algorithms, banks can handle a large volume of customer inquiries in real-time. These AI-driven solutions can provide immediate responses, with 24/7 availability, and personalized interactions which can enhance overall customer experience.
AI can also analyze vast amounts of customer data to personalize communication strategies. Machine learning algorithms can segment customers based on their preferences, behavior patterns, and transaction history. This segmentation allows banks to tailor marketing messages, product recommendations, and service offerings to meet individual needs effectively. Personalized communication not only improves customer satisfaction but also boosts engagement, loyalty, and retention rates.
In addition to streamlining customer interactions, AI can optimize communication channels for efficiency. For example, AI can prioritize and route customer inquiries to the most appropriate channels based on complexity, urgency, and customer preferences. This ensures that customers receive timely and relevant assistance, reducing wait times and enhancing overall responsiveness.
-
What are the key privacy and protection considerations for financial institutions when using AI?
Privacy and data protection are paramount considerations for financial institutions leveraging AI technologies. The sensitive nature of financial data requires robust safeguards to prevent unauthorized access, data breaches, and misuse. Key considerations include:
- Data Encryption: Implementing strong encryption protocols to protect data at rest and in transit, ensuring that sensitive information remains secure and confidential.
- Access Controls: Enforcing strict access controls and authentication mechanisms to limit data access to authorized personnel only, reducing the risk of unauthorized data exposure.
- Data Anonymization: Utilizing techniques such as data anonymization and pseudonymization to de-identify personal information, thereby safeguarding customer privacy while retaining data utility for analysis and AI model training.
- Consent Management: Obtaining explicit consent from customers for data collection, processing, and sharing purposes.
- Regular Audits and Monitoring: Conducting regular audits, vulnerability assessments, and monitoring activities to detect and mitigate security risks, anomalies, and potential data breaches proactively.
- Vendor Management: Implementing rigorous vendor management practices to ensure that third-party AI providers comply with security standards, data protection regulations, and contractual obligations.
Additionally, financial institutions must prioritize ethical AI principles, including fairness, transparency, accountability, and explainability. By integrating privacy-by-design principles and ethical AI frameworks into their AI initiatives, banks can address trust with customers, regulators, and stakeholders while mitigating legal, reputational, and operational risks associated with AI use.
-
Why is it important to train and test models to remove bias and ensure ethical use?
Training and testing AI models to remove bias and ensure ethical use are critical steps in responsible AI deployment for financial institutions. Bias in AI systems can lead to unfair treatment, discrimination, and unintended consequences, undermining trust, and credibility. Therefore, addressing bias and promoting ethical use are essential for several reasons:
- Fairness and Equity: Removing bias ensures that AI systems treat all individuals fairly and equitably, regardless of demographic factors such as race, gender, age, or socioeconomic status. Fairness promotes inclusivity, diversity, and equal opportunities in decision-making processes.
- Compliance and Risk Mitigation: Compliance with regulatory requirements and industry standards regarding fairness, non-discrimination, and ethical AI practices is crucial for financial institutions. Failure to address bias can result in legal liabilities, regulatory fines, and reputational damage.
- Customer Trust and Satisfaction: Ethical AI practices, including bias mitigation, transparency, and explainability, enhance customer trust, satisfaction, and loyalty. Customers are more likely to engage with AI-powered services and products that they perceive as fair, transparent, and accountable.
- Reputation and Brand Integrity: Demonstrating a commitment to ethical AI principles and responsible data use strengthens a bank’s reputation, brand integrity, and market competitiveness. Ethical considerations are increasingly important in shaping public perceptions and consumer preferences regarding AI technologies.
To remove bias and ensure ethical use, various strategies can be used, including:
- Diverse and Representative Data: Using diverse and representative datasets to train AI models reduces bias and improves model fairness. Data collection practices should prioritize inclusivity and avoid reinforcing existing biases.
- Fairness Metrics: Incorporating fairness metrics, such as disparate impact analysis, equal opportunity metrics, and demographic parity, during model development and evaluation helps quantify and address bias.
- Bias Audits and Testing: Conducting bias audits, sensitivity analyses, and robust testing procedures across different demographic groups and use cases helps identify and mitigate bias effectively.
- Explainable AI: Implementing explainable AI techniques, such as model interpretability methods and transparency mechanisms, enables stakeholders to understand how AI decisions are made and detect bias patterns.
- Continuous Monitoring and Feedback: Regularly monitoring model performance, analyzing outcomes, and soliciting feedback from diverse stakeholders facilitate ongoing bias detection, mitigation, and improvement efforts.
Financial institutions can uphold integrity, fairness, and trustworthiness in AI-driven decision-making by integrating bias mitigation strategies, ethical AI principles, and continuous improvement processes into their AI governance frameworks.
-
How can financial institutions effectively control toxic information from models?
Controlling toxic information from AI models involves implementing robust governance frameworks, advanced filtering techniques, and proactive monitoring strategies. Toxic information, such as hate speech, misinformation, or offensive content, can have detrimental effects on users, communities, and organizational reputations. Therefore, financial institutions must take proactive measures to identify, mitigate, and prevent the dissemination of toxic information from models. Key strategies include:
- Governance and Policies: Establishing clear policies, guidelines, and codes of conduct regarding acceptable content, prohibited behaviors, and responsible use of AI-driven platforms. Governance frameworks should outline accountability mechanisms, escalation procedures, and enforcement actions for handling toxic information incidents.
- Content Moderation Tools: Leveraging AI-driven content moderation tools, natural language processing (NLP) algorithms, and sentiment analysis techniques to detect and filter out toxic content in real-time. These tools can identify language patterns, contextual cues, and behavioral signals associated with toxic information, enabling proactive moderation and intervention.
- User Reporting and Feedback: Implementing user reporting mechanisms, flagging systems, and feedback loops that empower users to report toxic content, provide context, and contribute to content moderation efforts. User feedback helps prioritize moderation tasks, improve algorithmic accuracy, and address emerging content risks.
- Human Oversight and Review: Augmenting AI-based moderation with human oversight, manual reviews, and expert judgments to assess nuanced content, contextual nuances, and complex situations that require human judgment. Human moderators can identify subtle forms of toxicity, interpret cultural nuances, and apply context-specific rules to enhance content moderation accuracy.
- Collaboration and Industry Standards: Collaborating with industry peers, technology partners, researchers, etc.
-
How can financial institutions ensure models produce fair and unbiased output?
Financial institutions can ensure models produce fair and unbiased output by adopting a holistic approach that encompasses data collection, model development, and post-deployment monitoring. This includes:
-
- Collecting diverse and representative datasets to mitigate biases.
- Incorporating fairness metrics and conducting bias audits during model development.
- Implementing explainable AI techniques to understand model decisions and detect biases.
- Regularly monitoring model performance, analyzing outcomes across different demographic groups, and promptly addressing any disparities. By prioritizing fairness and transparency throughout the AI lifecycle, financial institutions can foster trust, mitigate risks, and promote ethical use of AI.