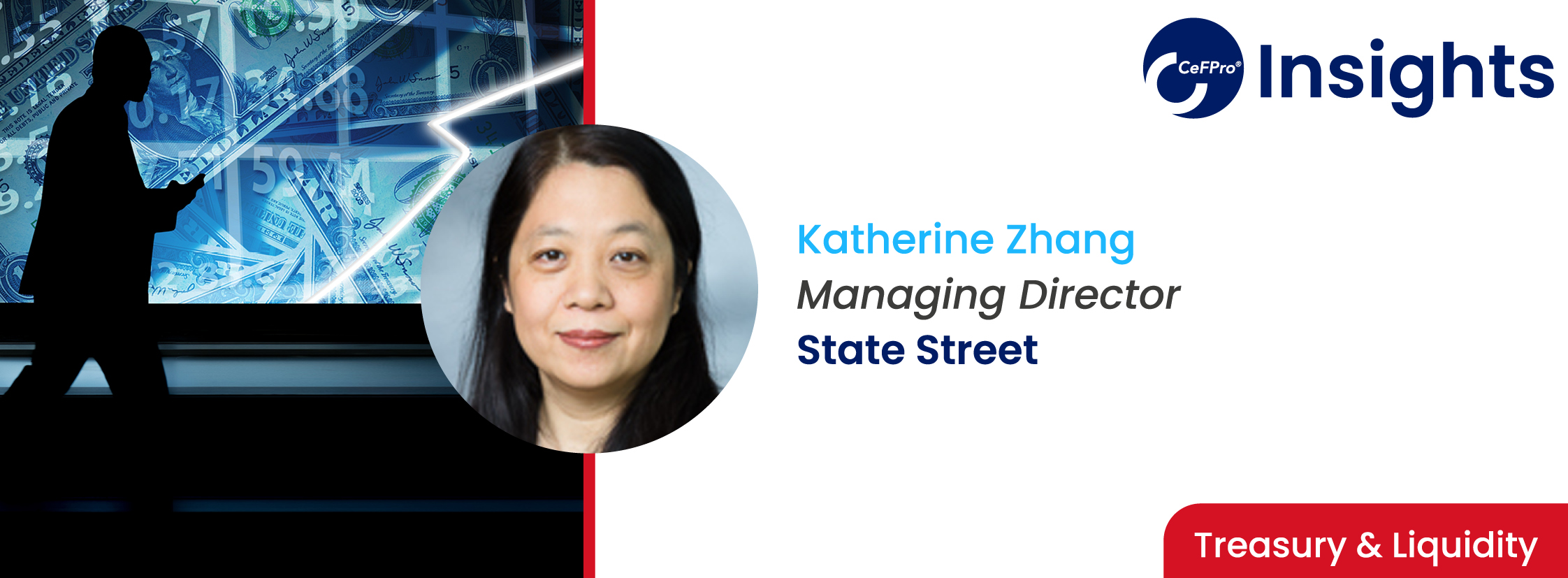
Forecasting deposits in an uncertain macroeconomic environment
Katherine Zhang, Managing Director, State Street
Below is an insight into what can be expected from Katherine’s participation at CeFPro’s Balance Sheet Management USA Congress.
The views and opinions expressed in this article are those of the thought leader as an individual, and are not attributed to CeFPro or any particular organization.
How do you factor in behavioral aspects when forecasting deposits?
Deposit forecasting is challenging because it requires factoring into the dynamics between bank and client behaviors. Non-maturity Deposits (NMDs) are complex financial instruments that include embedded options for both the bank and customers. Banks have the option to adjust interest rates, while customers have the option to withdraw funds. Failing to understand and account for these options can lead to ineffective modeling and risk management
From the client behavior perspective, segmentation is one of the key aspects when forecasting deposits. In addition, the model ideally should be both retrospective and prospective. Retrospective requires a bank to define the core based on the history and separate the core from surging balances in each sector. This is because the surge balances will leave the institution during economic booming/recovery and flow in during recessions, impacting cash flow assumptions. Prospective requires a bank to adjust the core based on expectations from management, e.g., flight to quality, rate differentials, technology, demographics, and competition.
How can deposit modeling be effectively incorporated to manage liquidity and interest rate risk within a financial institution?
The deposits model should be designed to capture the dynamics of clients’ deposits from short-term and long-term separately for liquidity and interest rate risk purposes. The liquidity risk focuses on the client’s behaviors from 30 days to one year under either BAU or stress scenarios. In contrast, the interest rate risk should be articulated from both the economic value of equity and earnings in the short or midterm (two to three years). The duration of deposits and a financial institution’s pricing strategy are generally the focus of deposit behavior studies, which are critical inputs to analyze the impact on NII and EVE. Finally, concentration risk should be quantified or measured, e.g., HHI, and contribute or be part of risk appetite or limits calibration.
How have models had to change for deposit pricing whilst there is an uncertain macroeconomic environment? Is this still an increasing risk?
The deterioration of the performance of the pricing model may lead to an inaccurate assessment of interest rate risk for the economic value of equity and a poor projection of NII outlook in the short and near term. The challenge of deposit pricing models is to ensure that a model can perform well throughout a full economic cycle, meaning under both high and low rate environments specifically. Per IRRBB requirement, EVE and earning-based risk measurement requires a bank to evaluate the interest rate risk under a wide and appropriate range of interest rate shock and stress scenarios, i.e., beyond the normal range of the historical rates observed recently.
Throughout the pandemic, the model limitation of deposit pricing has become elevated and probably more severe during the consecutive rate hikes by Fed started in March 2022. The historical data based modeling approach is likely grounded in the low-rate environment and therefore begged for upgrades or enhancements from both business and regulatory perspectives. For example, the betas for the higher rate buckets deviated from the recent business practice significantly in 2022. The remediation approaches could include weighting the recent data more heavily or adopting a more advanced statistical approach, e.g., an error correction model (ECM). The regime shift happened in 2022, i.e., the level of rates transitioned at an unprecedented speed from a low-rate environment to a high-rate environment. The merit of ECM is that it can estimate both short-term and long-term effects of one-time series on another. ECM can do a better job when you not only consider either just a long-term relationship or just a short-term relationship compared to other statistical approaches for time series data. Specifically, ECM describes how the dependent variable (y) and the independent variable (x) behave in the short run, consistent with a long-run cointegrating relationship. To elaborate, first, the long-term relationship between the co-integrated variables is captured by regressing y and x values. Then the error terms of this regression, together with other short-term drivers, is leveraged to correct the short-term trends, aligning with the long-term equilibrium. (Equilibrium reversion).
However, an interim solution may be needed before a good model can be built, validated, and put into production, given the uncertainty of macroeconomic conditions and the limited length of data under the high-rate environment. One solution could be to add an overlay on top of model output based on SME’s assumption incorporating a bank’s pricing strategy.
It is still an increasing risk due to the uncertainty of economic conditions and data limitations. Making the deposit pricing model more agile and frequently aligned with SME’s assumption or a bank’s pricing strategy would be desired and necessary.
Why is it important to model extreme deposit runoff overnight? What are the associated risks if this is not done?
A significant run-off overnight could cause a serious liquidity issue when alternative funding sources could not be leveraged or tapped into by a bank, or a bank becomes unable to meet the demand for withdrawals and insolvent, such as SVB where highly concentrated depositors withdrew their funds simultaneously within a short time window. An uncontrolled bank run can wipe out shareholders, bondholders, and depositors (beyond the insured amount). As multiple banks get involved, it could also spark a cascading industry-wide panic that can cause a more systemic financial crisis.