This content has been archived. It may no longer be relevant
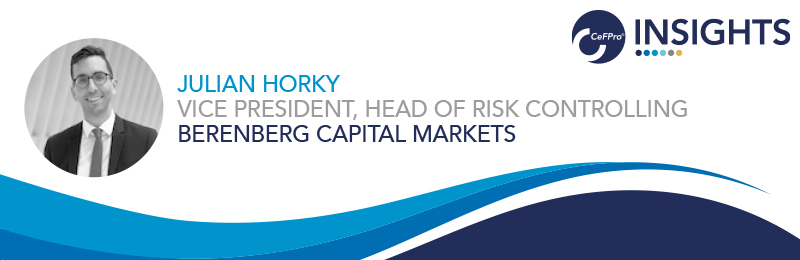
Implementing a Trade Surveillance Program to increase fraud detection
The views and opinions expressed in this article are those of the thought leaders as individuals, and are not attributed to CeFPro or any particular organization.
By Julian Horky, Vice President, Head of Risk Controlling, Berenberg Capital Markets
How does implementing a Trade Surveillance Program aid in increased fraud detection
A good trade surveillance program improves fraud detection while lowering costs. But why do businesses even struggle with fraud detection? More regulatory pressure and new fraud strategies challenge banks and require agile fraud detection processes. A common mistake is to throw bodies at these challenges. The latest regulations, such as MiFID II, MAR, and US Dodd-Frank, were countered with more compliance and risk staff. However, the situation is getting worse by the year as regulations keep getting more complex. Additionally, regulations will only get more rigorous in the next decades – just look at the previous five decades. As a result, building more agile fraud detection capabilities is a better alternative than raising compliance and risk costs every year.
A good trade surveillance program enables such enhanced fraud detection capabilities. Like sharpening an axe before chopping down trees, it makes every step that follows easier – whether it’s data gathering, model development, analysis, automation, controls, or everything else you may think of. Fraud detection processes that rest on a sound trade surveillance program are more effective, more efficient, and need less human intervention. So, in my opinion, a trade surveillance program is really about providing the foundation and the framework to enable simple, effective, and agile fraud detection.
Has Covid-19 affected how effectively an organization can detect fraud?
Yes, it certainly has – and there’s a strong consensus across the industry. Threats evolved as a result of the coronavirus. The rate at which this occurred put regulators, market participants, and myself in awe. Overall, it made fraud detection more important and simultaneously more difficult. When market activity soared at the beginning of corona, it was coupled with more fraud and, at the same time, also more false alarms. This is remarkable: more fraud and more false alarms. How is that possible?
Market volatility in February and March was accompanied by more activity – precisely as one would expect. But many fraud detection systems were caught off-guard by the sudden shift in behavioral patterns. As a result, practitioners and regulators witnessed many so-called “false positives” – the algorithms flagged innocent activities as fraudulent. Trade surveillance and AML programs react sensitively to more false positives as it requires more human interventions. As a result, many algorithms developed with old datasets needed to be adjusted to reflect the change in the environment to remain effective.
What is the role of AI in trade surveillance?
We are at a fascinating point in the evolution of artificial intelligence and trade surveillance. As we speak, it is already widely utilized, but even more is on the way. In fact, AI is already being heavily used for trade surveillance – and it has been for a while. I remember how early the AML and trade surveillance community adopted it and until this day, it continues to produce spectacular results. These results are much better than more traditional rule-based algorithms – often by magnitudes. They outperform rule-based algorithms in accuracy and breadth, with the semi and unsupervised algorithms really sticking out. They don’t just outperform: AI algorithms also enable new applications that wouldn’t have been possible in the past. (Think – natural language detection.) You can clearly find AI along the whole process chain, which includes getting the data, aggregating it, developing, validating and running the models and of course generating alerts with these models.
One disadvantage of AI algorithms is their black-box nature, which makes it difficult for business and compliance to understand what’s going on behind the scenes. Regulators across jurisdictions continue to highlight the need for algorithms to be comprehensive, and model risk management groups are catching up quickly.
As the use of AI grows in popularity, has this changed the landscape and future of how we detect fraud?
Across the industry, it is almost universally understood AI will ultimately take over fraud detection – I doubt many practitioners disagree. What’s still very opaque is the question of timing — namely, when this will happen. New technologies have this hype cycle characteristic that they are often exciting but may underdeliver in terms of timing. AI could be one of them. Everyone agrees that AI will eventually take over fraud detection, but whether it will be in 5 years or 25 years – we just don’t know yet.
However, we do know what the near future of fraud detection will look like. I expect to see more and more unstructured data use cases, as we did in the last decade. Connecting disparate data sets will reveal previously unseen insights – the unknown unknowns. This includes emails or phone conversations but also metadata and digital behavior.
With all this technology, the question is where to draw the line: where do we stop? From an economic perspective, the answer is simple – detection costs and detection benefits constitute the optimum level of fraud detection. It’s not as clear in terms of other conflicting goals such as privacy. Fraud detection may interfere with privacy – a topic that is of growing concern for legislative bodies; think of the latest GDPR rules in the EU.
What are some common frauds and detection methods used for this?
The universe of fraud is endless. Some frauds are extremely simple and mundane, while others are complex mechanisms that leave you behind in open-mouthed astonishment.
Insider trading is probably one of the more prevalent white-collar crimes, occasionally making it into the mainstream media. I recall the Martha Stewart case and, more recently, the 2020 congressional insider trading allegations. A common way to identify insider trades is by looking for particularly favorable trades that were followed by news media articles right after the trade. While the detection method may appear simple, it requires natural language processing, dependable data sources, and sound calibration. The algorithm must not flag too many legitimate trades while still detecting the fraudulent ones.
Spoofing is another form of fraud, and it is often tied to the flash crash of 2010, where a young trader caused one of the fastest crashes in recent history orchestrated from his parents’ house. It has also received increased public attention during corona due to Robinhood, and meme stocks like GameStop. Spoofing is a practice in which orders are placed and canceled quickly to shift prices. At the same time, an actual trade is executed on the opposite side of the book. For example, a fraudulent buy order is placed for fractions of a second and drives up the price up. The prospective spoofer exploits this through a sell. Among all algorithms that are used to detect spoofing, many are based on monitoring the opposite sides of the book, for example, reviewing the opposite side of the book for each fill.