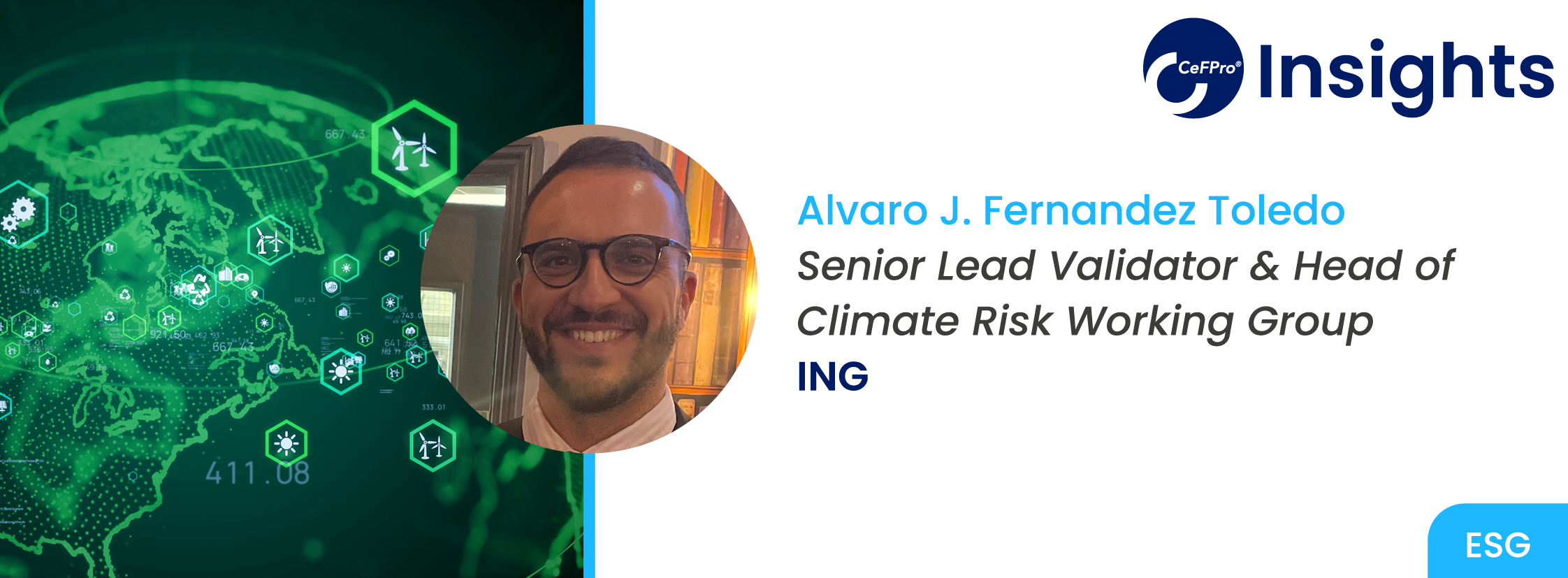
Developing innovative methods to incorporate physical and transition risks within stress testing
Alvaro J. Fernandez Toledo, Sr Lead Validator & Head of Climate Risk Working Group, ING
Below is an insight into what can be expected from Alvaro’s session at Climate Stress Testing 2023.
The views and opinions expressed in this article are those of the thought leader as an individual, and are not attributed to CeFPro or any particular organization.
Why is it important to incorporate physical and transitional risks within stress testing? Should these two climate risks be incorporated?
It is important to include these risks in internal or regulatory stress testing exercises since the impact could be extremely high based on climate models that have been published by physicists and experts (e.g., NGFS or IPCC). Therefore, the fact of avoiding physical and transition risks in stress testing implies the worst-case scenario in risk management: risks are not properly identified nor quantified. Even though we can define these two kinds of risks (physical and transition) within climate stress testing, they are intertwined. It is impossible to isolate any of these two risks because it means an implicit assumption on the other risk. No actions to transition to a Low Carbon Economy (LCE) in terms of policy action, technological development, or market sentiment/confidence shock means that there is no transition risk; however, in this scenario, all the downside effects and risks will come from climate change. Equivalently, a disorderly and sharp transition to an LCE means that there is a high transition risk but low effects in the long term from physical risk events (acute and chronic). Therefore, if we want to perform a realistic and meaningful stress test exercise, we need to include both risks as well as dependencies. Direct effects are important, but also indirect and second-round effects since business models of companies will try to adapt and survive in the new hypothetical scenarios. In my opinion, this is the most complicated topic in climate risk stress testing since the variability and uncertainty in the dynamic portfolios of banks are quite high, especially considering that the forecast horizon can be defined up to 2050 or 2100. Stress Test models are well-known and useful for business strategy (3-5 years) but it is likely that traditional techniques will fail if no specific climate adjustments are introduced.
What are the key takeaways from DNB and ECB’s approaches to physical and transition risk?
In general terms, most papers that are currently available prove that the additional costs and losses that should be faced in the short term (in order to transition to an LCE) are much lower than the long-term losses that may be produced by climate change. Researchers have proved that the cumulative losses (e.g., cumulative PD) are always lower in the orderly transition scenario than in the disorderly transition scenario, and finally, the worst case is the hothouse world scenario. While there are differences depending on region, sector, and type of portfolios (retail vs. non-retail), it is important to convince society that global action is required. Local solutions will be inefficient and can generate frustration among people as well as disputes between countries/regions. I believe taking action based on science with a generous and realistic mindset is critical.
How do the top-down and bottom-up approaches differ when it comes to addressing transition risk?
As is known from traditional stress testing techniques, top-down approaches are adequate to estimate portfolio trends and sensitivity. However, bottom-up approaches are more granular, meaning that, in general, they have better discriminatory power as well as better adaptation to dynamic shifts. As previously mentioned, indirect effects are extremely relevant for a proper climate risk stress test. Therefore, bottom-up approaches seem to be more appropriate for this goal as well as to incorporate dynamic strategies in the economy. This can be integrated via risk driver information of each client, such as GHG emissions. However, top-down approaches are simpler, easier to understand, and useful for initial or temporary estimations. In this top-down approach, information at the client level is not used.
How can forecasting capabilities be developed when there’s limited historical data available?
It is true that anything related to ESG topics (ratings, GHG emissions, stress tests, IRB, etc.) is criticized because there is a lack of data, standardization, and many regulations (which are sometimes not aligned). In my opinion, we need to accept that this is the reality and will not change in the short term. Therefore, we need to be proactive and find creative solutions. Physicists have proved that we have observed almost nothing/too little about climate change. Therefore, we need to understand that all historical climate correlations and observations are outside the range of what could happen in the future, and more importantly, they are not representative. We should change our mindset of trying to predict losses merely based on historical events (such as in traditional credit risk models). Instead, we should be aligned with climate models that aim to predict what is going to happen in the future, with projections outside the range observed in historical data. The fact that future projections are outside the range of historical data is one of the main challenges in developing a proper climate risk model, and this also creates challenges for other areas, such as finding appropriate validation techniques for these types of models. Climate papers have shown that theoretical/mathematical/sensitivity analysis and benchmarking are the most accurate techniques to assess these models rather than relying exclusively on backtesting results. It is important to mention that current climate risk models that are published by central banks and authorities have a low accuracy based on historical samples (e.g., R2 of ECB PD Top Down Climate Risk Stress Test model is 11.9%). However, this observation does not necessarily imply that these models are incorrect in estimating future climate risks. There is a lot of knowledge out there (e.g., prediction of unobserved events in Physics) that we as bankers can learn from in order to incorporate climate risk into our stress testing frameworks.
What is the importance of developing a robust scenario formulation process?
The key aspect of defining a robust scenario analysis is to use realistic/feasible scenarios but also to understand what assumptions and limitations are behind these scenarios fully. For example, NiGeM, which is one of the most famous models from NGFS, has a mathematical methodology so much more complex than traditional credit risk models. Therefore, if we want to use these kinds of scenarios in stress testing, we need to understand the main assumptions of these climate-econometric models since we will use their outputs as our inputs.
Finally, I would like to mention that the climate concept of RCPs could be considered for internal and strategic purposes. This is defined as the multi-median model due to the high uncertainty even for specific scenarios (e.g., NDCs or orderly 1.5 Celsius degree). I consider it relevant to include this concept in our stress testing framework as well as sensitivity analysis (for example, via the percentiles 10-90). If only one model forecast is used to define these scenarios, there is a high probability of not being representative of the rest. Climate experts consider that the RCPs are the best estimation for global forecasts (from Global Climate Models – GCM), while Regional Climate Models (RCM) are considered more accurate for local purposes. We should follow the corresponding approach depending on the regions we are considering in the scope.
Since climate events’ effects on the economy and society are quite complex, expert knowledge is needed to perform internal predictions (such as ICAAP).