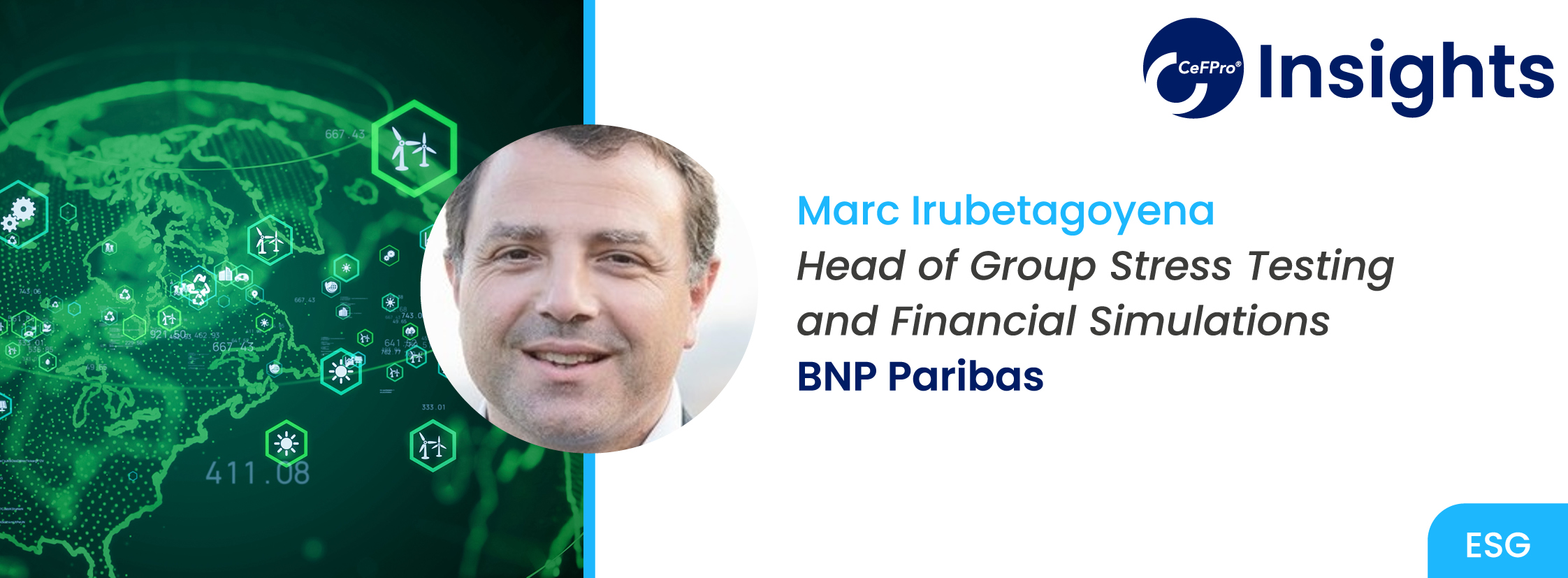
Managing complex data requirements to incorporate climate into stress testing models
Marc Irubetagoyena, Head of Group Stress Testing and Financial Simulations, BNP Paribas
Below is an insight into what can be expected from Marc’s session at Climate Stress Testing Forum Europe 2023.
The views and opinions expressed in this article are those of the thought leader as an individual, and are not attributed to CeFPro or any particular organization.
Why is it challenging to manage complex data requirements when incorporating climate into stress testing models?
Climate risk factors extend the data list necessary for stress testing models on a wide range of new types of data (asset localization, type of asset, protections of the asset, Energetic Performance of the asset, role of the asset in the production chain, GHG emissions, transition plans of the companies, energy consumptions, …). The list is getting longer each time modeling is going one step beyond in the analysis.
The key challenge is to be in a position to prioritize the data collection since the data roadmaps of the financial institutions are already quite loaded by the pace of regulatory and supervisory evolutions. Prioritization demands to have a risk assessment in place to highlight critical information versus less informative data. It is a process of iterative analysis between data acquisition and modeling outcomes that is needed. Not an easy task!
What are the most complex data requirements to adhere to when incorporating climate-related factors into stress testing models?
As of today, information will be progressively gathered either by direct data collection between clients and the institutions or through third party data collection. The hardest data requirements will remain on the evolution undergone by the clients in terms of adaptation of their production and supply chains. Public commitments will be used, but they will often remain at a business group level and not necessarily at the counterparty level of a financial institution. Most likely, they will not cover the full spectrum of critical adaptation questions.
What are some difficulties with building reliable models when there is limited historical data available?
Climate scenario analysis is creating a significant challenge for the design of reliable models. Beyond traditional statistical approaches, the modeling will require adding forward-looking layers to capture impact chains between new transmission channels and the risk drivers of financial risks, such as credit, market, operational, and financial risks. A hybrid modeling framework combining regulatory and forward-looking models will also demand greater proximity between the various modeling teams to enhance anticipation and efficiency of the deliveries. This represents a cultural change with new ways of working.
What are the best strategies or methods you have seen to convert climate data into financial data?
Risk assessment and risk identification are the grounding steps of climate scenario analysis. They target notably to detect the most exposed perimeters and to identify most material risk factors associated with climate change for the financial risks of the institutions. The next step in the process is to understand precisely the most relevant transmission channels between material risk factors and the considered financial risks of the institutions (prices of various energies, for example, as a transmission channel between climate risk transition factors and financial ratios involved in credit risk measurement). Using climate evolution scenarios, with all the extensions of forward-looking evolutions of economic variables, risk drivers can be projected by identifying transmission channels. Obviously, this is an iterative process that will keep on being updated going forward in the transition roadmap.
Why is it important to have granular data to ensure better decision-making across portfolios and clients?
To run risk measurement, it is possible for some data to use shortcutting approaches in case of missing or improper data. Obviously, this demands additional work to assess the uncertainty in the modeling created by these shortcuts and also check that the best shortcutting approach has been retained. Work is ongoing also between banks to share shortcutting practices to avoid discrepancies in the assessments purely due to shortcutting diverging practices.
Acceptable shortcuts for portfolio management can raise concerns when looking at a single counterparty or transaction. Additional adaptation of the data collection and the modeling can be required when getting to individual transaction decision-making. There again, an iterative learning process has to be put in place between bottom-up and top-down risk analysis.