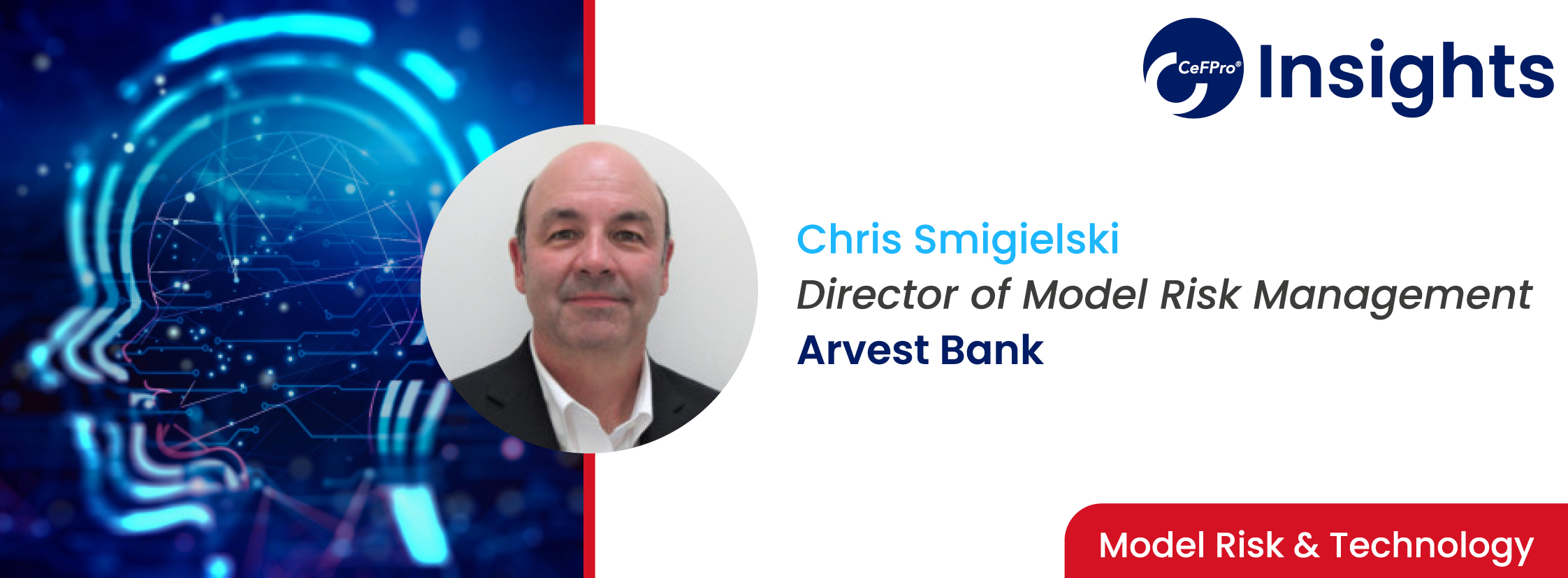
Managing complexities with the ongoing expansion of model risk scope and inventory
Chris Smigielski, Director of Model Risk Management, Arvest Bank
Below is an insight into what can be expected from Chris’s session at Advanced Model Risk USA 2024
The views and opinions expressed in this article are those of the thought leader as an individual, and are not attributed to CeFPro or any particular organization.
Do financial institutions need to enhance MRM programs to accommodate AI?
With digital transformation driving an evolution across financial services, the integration of Artificial Intelligence (AI) into various facets of operations has become both a necessity and a challenge for financial institutions. As AI continues to permeate modeling activities, the need to adapt and enhance Model Risk Management (MRM) programs has emerged as a critical priority. While AI promises enhanced predictive capabilities and efficiency, its inherent complexity and unique characteristics demand a tailored approach within MRM frameworks.
One of the primary requisites for accommodating AI within MRM programs is the cultivation of specialized expertise. Building multidisciplinary teams proficient in both AI and traditional modeling is indispensable. These teams require a deep understanding of AI algorithms, their limitations, and their application in financial and operational models to effectively manage the risks associated with their utilization.
Validation methodologies within MRM frameworks should evolve to address the idiosyncrasies of AI models. Specific validation processes must be designed to address the non-linearity, complexity, and lack of transparency often inherent in AI-driven models. Ensuring the explainability and interpretability of AI models is a requirement, allowing stakeholders to explain the decision-making processes while complying with regulatory expectations. As AI introduces potential biases and ethical dilemmas, robust frameworks must be established to ensure adherence to regulatory standards and ethical guidelines.
Dynamic monitoring and governance mechanisms are another crucial enhancement necessary to effectively manage AI models within MRM programs. Unlike traditional models that operate within predefined boundaries, AI models exhibit adaptive behavior and necessitate continuous monitoring to detect model drift and trigger interventions as needed.
Collaboration between MRM, data governance, and data science teams becomes imperative for seamless integration, standardized data governance, and the establishment of ethical AI use for the organization. This collaboration enables a comprehensive understanding of AI models’ function, data sources, and performance metrics.
Comprehensive documentation and reporting standards tailored for nuances of AI models are essential components of enhanced MRM programs. These standards encompass detailed documentation of model assumptions, data sources, and performance metrics, ensuring transparency and accountability.
By embracing specialized expertise, rigorous validation, dynamic monitoring, ethical compliance, and collaborative efforts, financial institutions can navigate the complexities of AI models, ensuring robust risk management and regulatory compliance in an ever-evolving landscape.
Does Generative AI belong in the model inventory?
Generative AI (e.g., ChatGPT, DALL.E, Bard, etc.) can be classified as a model under MRM guidance (SR 11-7/OCC 2011-12) based on several key elements that align with the definition and characteristics outlined in the regulation:
- Inputs and Outputs: Generative AI operates based on inputs (training data) and produces outputs (generated content) based on learned patterns. These inputs and outputs align with the model definition, where a model is broadly defined as any quantitative method, system, or approach that applies statistical, economic, financial, or mathematical theories.
- Complex Algorithms and Methodologies: Generative AI utilizes complex algorithms, frequently based on deep learning techniques. These algorithms learn patterns from data and generate new content, meeting the description of a model.
- Risk Management Considerations: Generative AI poses risks related to data quality, biases, ethical considerations, and operational challenges, necessitating risk management procedures similar to those for traditional models.
- Validation and Testing: MRM guidance highlights the need for robust validation and testing for models. Generative AI models require thorough validation to ensure accuracy, reliability, and adherence to ethical standards. This involves validating outputs, assessing biases, and ensuring the model’s suitability for its intended use.
In essence, generative AI meets the criteria outlined in MRM guidance as it embodies the fundamental characteristics of a model. Its complexity, reliance on algorithms, inputs, outputs, risk implications, and validation requirements align with the principles and expectations presented in MRM guidance.
Why is it important for financial institutions to consider developing replacements for an aging inventory using newer methods like AI?
The evolution of banking and finance has been accompanied by a constant demand for more accurate, efficient, and adaptable models to manage risks, make informed decisions, and meet regulatory requirements. As technology advances, banks face the imperative need to replace older/legacy models with newer, more sophisticated models and model components with methods including Artificial Intelligence (AI). This transition is crucial for several reasons, outlining the importance of potentially embracing AI as a replacement for traditional models.
First, the pace of change in financial markets demands models that can swiftly adapt to evolving dynamics. Older models might not perform well with the complexities and volume of data generated in today’s interconnected and fast-paced financial ecosystem. AI, with its ability to process vast amounts of data and detect intricate patterns, presents a compelling solution to this challenge. It enables banks to build models that can analyze data in real-time, providing more accurate and timely insights into market trends and risks. AI is not an ‘instant fix’ to all modeling problems, but in many cases, it can produce a better outcome.
Second, traditional models often have limitations in handling non-linear relationships and complex interactions within financial data. AI-based models, such as neural networks or deep learning algorithms, excel in capturing these intricate relationships. They can uncover hidden correlations, mitigate the risk of oversimplified assumptions, and enhance predictive accuracy.
Moreover, the competitive landscape in the financial sector is constantly evolving. Banks that leverage AI to replace outdated models gain a competitive edge by harnessing technology to offer innovative products and services. AI-driven models enable banks to personalize customer experiences, optimize operations, and develop sophisticated risk management strategies, thereby improving their market position and customer satisfaction.
The adoption of AI in replacing older models, however, is not without challenges. Banks must address concerns related to model interpretability, ethical considerations, and robustness. Ensuring that AI models are explainable and transparent is crucial for gaining regulatory approval and maintaining trust among stakeholders. The ethical implications of AI, such as bias in algorithms or data privacy concerns, demand careful consideration and mitigation strategies, but in the long run, may improve modeling outcomes.
How can financial institutions effectively address Enterprise AI governance?
The advent of Artificial Intelligence (AI) within financial services has revolutionized the landscape, offering transformative capabilities to streamline operations, enhance decision-making, and improve customer experiences. However, with the integration of AI across diverse functions, the complexity of managing risks extends beyond Model Risk Management (MRM) to encompass a spectrum of broader enterprise-level challenges. Addressing these risks necessitates a comprehensive approach to AI governance that spans the entire organization.
Establishing clear governance frameworks forms the bedrock of effective enterprise AI governance. These frameworks outline policies, protocols, and guidelines governing AI adoption, ensuring alignment with ethical, legal, and regulatory standards. By defining responsible AI principles, institutions commit to fostering fairness, transparency, and accountability in the development, deployment, and utilization of AI systems.
Central to robust AI governance is a focus on data governance and management. Financial institutions must enforce stringent data governance practices to ensure the integrity, quality, and privacy of data used by AI systems. Adherence to strict protocols for data collection, storage, processing, and sharing, coupled with compliance with privacy regulations, safeguards against data-related risks.
Transparency and explainability are foundational pillars in the governance of AI systems. Financial institutions must ensure that AI-driven decisions are comprehensible and justifiable. This transparency fosters trust among stakeholders and regulators, particularly in sensitive areas like customer interactions and financial decision-making.
Continuous monitoring and evaluation mechanisms are indispensable to track the performance, accuracy, and fairness of AI systems. Rigorous assessments for biases, deviations, or shifts in performance enable proactive intervention and ensure ongoing compliance with ethical and regulatory standards.
Cross-functional collaboration plays a pivotal role in effective AI governance. Encouraging interdisciplinary dialogue among IT, risk management, compliance, legal, and business units facilitates a holistic approach to addressing AI-related challenges.
Staying abreast of evolving regulatory requirements is important as well. Financial institutions must ensure compliance with a dynamic regulatory landscape, adapting governance practices accordingly to mitigate legal and compliance risks.
Board and senior management oversight are instrumental in steering effective AI governance. Active involvement in decision-making and risk management strategies, coupled with a deep understanding of AI-related risks, reinforces the institution’s commitment to responsible AI utilization. In that effort, ethical review boards or committees serve as guardians of ethical standards, ensuring alignment of AI applications according to the board’s intentions and the institution’s values. These entities are entrusted with reviewing the ethical implications of AI implementations, reinforcing ethical practices throughout the organization.
Comprehensive enterprise AI governance transcends conventional model risk management, encompassing a multifaceted approach to address risks across the AI lifecycle. By adhering to robust governance frameworks, emphasizing data governance, transparency, continuous monitoring, regulatory compliance, and ethical considerations, financial institutions can navigate the complexities of AI adoption while ensuring responsible, ethical, and secure utilization of AI systems across the organization.
How can financial institutions utilise the OCC’s 8 categories of risk to effectively manage model governance and inventory?
The Office of the Comptroller of the Currency’s (OCC) Comptroller’s Handbook booklet, “Model Risk Management,” (August 2021) mentions eight categories of risk commonly encountered by financial institutions: credit risk, interest rate risk, liquidity risk, price risk, operational risk, compliance risk, strategic risk, and reputation risk. Models play a significant role in each of these categories and require lifecycle governance to manage the associated risks effectively.
Generally, the early implementation of model risk management guidance (SR 11-7, OCC 2011-12, etc.) focused governance over financial models because of their direct impact on financial statements and management decision-making. Model inventory’s have broadened to include much more than just financial models due to digital transformation; the ramp-up and use of AI & machine learning modeling methodologies. Recognizing that models may produce an estimate is only the beginning of an assessment that should include documenting and testing for data bias, algorithmic bias, and related tangential risks; for example, reputational damage or strategic risks depending on the third/fourth party handling of data and methodology implications to accomplish the initial modeling objective. Robust validation, continuous monitoring, and transparent documentation help to ensure accuracy, reliability, and adherence to regulatory requirements. Simply executing traditional MRM frameworks may not be enough to capture risks or interdependencies that span across the risk appetite. Collaboration with other risk areas such as Compliance can help drive a broader conversation and comprehensive risk assessment. Gap analysis can then highlight where exposures exist and control frameworks can be updated accordingly.
Adhering to these governance practices ensures that model risks across these categories are identified and controlled to maintain regulatory compliance, accuracy, and transparency in decision-making. This approach ensures a holistic and structured framework to govern AI models, enabling institutions to capitalize on the benefits of AI while safeguarding against potential risks.