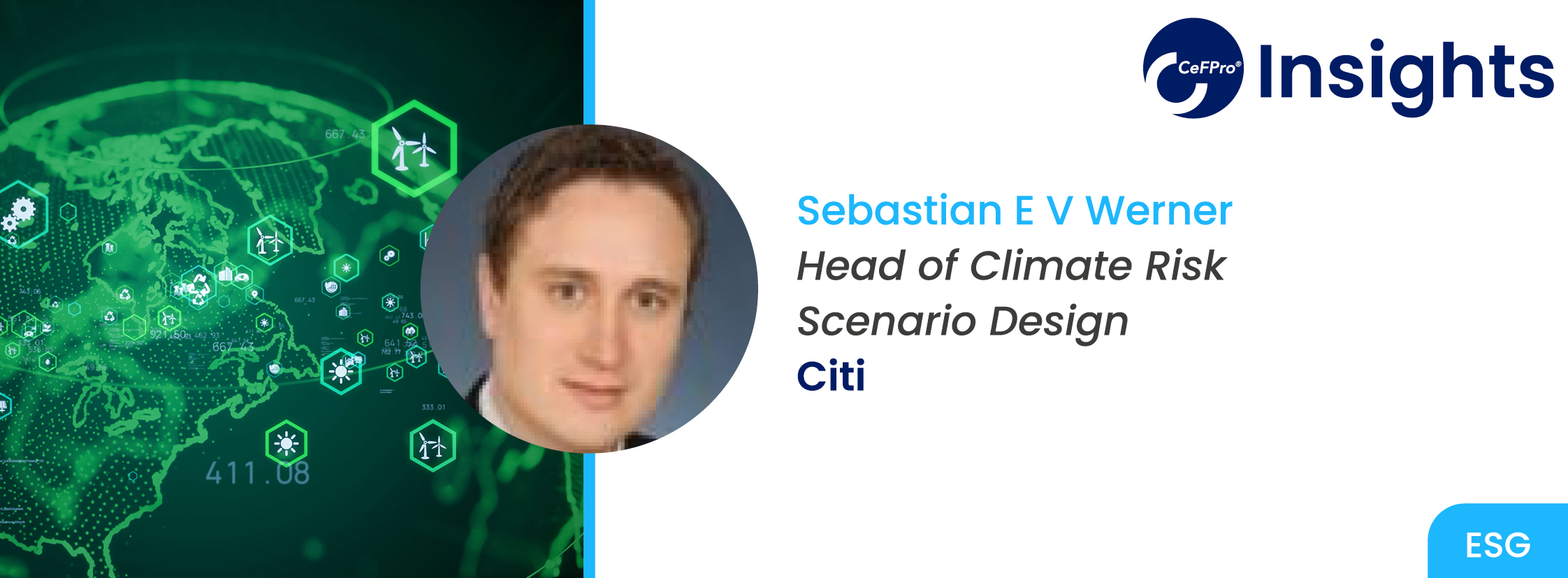
Managing complexity of modeling requirements with longer time horizons for climate scenarios
Sebastian E V Werner, Head of Climate Risk Scenario Design, Citi
Below is an insight into what can be expected from Sebastian’s session at Climate Stress Testing Forum Europe 2023.
The views and opinions expressed in this article are those of the thought leader as an individual, and are not attributed to CeFPro or any particular organization.
In what ways do longer time horizons for climate scenarios impact modeling requirements?
Traditional stress testing (CCAR, EBA, PRA, etc) usually assumes a scenario horizon of 3 to 5 years, whereas long-term climate scenarios usually end in 2050 (net zero target). Shorter regulatory scenarios focus on the current state of the business cycle. In the case of an economic shock, severe corrections across various markets and a subsequent decline in the real economy are simulated. Whereas signal and cycle are the predominant factors in the traditional scenario, trend assumptions are a scenario afterthought and are usually assumed to grow at a constant rate; the reverse holds in most climate scenarios: The trend is the dominant feature of the scenario, and its growth rate varies across time points. The cycle only determines the starting point; any signal can be discounted as noise. This requires a substantial rethink of scenario model dynamics: Long-term productivity and population growth determine gross domestic product (GDP). Inflation spikes within quarters make way for the long-term inflation trend across several decades. Long-run economic growth and trend inflation determine the steady-state level of interest rates. Whereas cyclical unemployment is largely driven by the dynamics of recessions and expansions (business cycles), climate scenario modeling should explain any changes in the natural unemployment rate (also called the structural unemployment rate): Does a higher wet bulb temperature lower labor productivity? How does a reduced oil/gas demand impact the local labor market of fossil-fuel producing county, state, or even country?
What are the key benefits of merging climate science and economics to address global environmental challenges?
It has long been recognized that climate change poses a major problem in the economic sciences as acute and chronic damages impact economic activity. On the other hand, economic activities determine the level of greenhouse gas (GHG) emission and, ultimately, the speed and direction of atmospheric changes. Merging both disciplines offers at least three key benefits. First, it helps us assess the economic impact of acute and chronic damages and the transition from fossil fuels. For example, extreme weather events such as hurricanes severely disturb economic activity by destroying vital infrastructure. Rigorous econometric modeling can map the response physical infrastructure damage has on economic activity. Discount pricing models can incorporate long-term sea-level rises into today’s coastal house price indices. Integrated assessment models combine macroeconomic dynamics with energy pathways and predict the speed of oil demand destruction. Secondly, the merging of climate and economics helps to efficiently allocate the necessary resources to adapt to climate change (greater climate resilience through improved energy efficiencies in residential and commercial buildings, planting trees to reduce heat, etc.) as well as mitigate climate change by reducing GHG through investment in renewable technologies and the investment in research and development in new technologies. Thirdly, it helps to translate climate uncertainty into economic uncertainty, ultimately determining potential mispricing in financial markets and greater risk aversion for investors.
What are the essential data requirements for conducting complex modeling, and how can firms ensure they meet them?
Unlike traditional scenario modeling, climate scenarios require much greater industry and geographic granularity. The required granularity is often more aggregate than the idiosyncratic risk of the asset itself. Moreover, historical series are difficult to obtain and may not be as useful for predicting future relationships. However, through a combination of company-specific metrics and industry benchmarks in the case of transition risk and a combination of obligor-specific locations and geo-specific perils in the case of physical risk, firms may meet these requirements sufficiently. For example, the emissions for any given industry are not necessarily representative of a specific company within the industry, and they may have dropped over time for reasons unrelated to any carbon price increases. Additional data requirements that would be essential are the company-specific emission-to-revenue ratio and benchmarking these against the industry trend.
What methodologies and data sources should be utilized when developing forward-looking models to forecast climate risks and their potential impacts?
Historic relationships are usually a poor guide to explaining climate change’s impact on the economy and financial markets. Extreme weather events that have not yet been observed (acute risk), temperature pathways hitting thresholds (chronic risk), and the pathway to carbon-friendly technologies (transition risk) will cause nonlinearities of unknown form in economic systems. To overcome the inherent nonlinearities, modelers should gather very granular data series to uncover relationships that are immune to nonlinearities and/or develop methodologies that are more qualitative and make outright assumptions about future relationships between climate-specific drivers and economic factors. Although integrated assessment models (IAM) have been used extensively in the climate change sciences, financial risk modelers should instead utilize the scenarios derived from the IAMs and then apply specific methodologies that translate energy pathways to financial metrics such as sector-specific gross value added, equity indices and corporate bond spreads.
Why is it essential to continually develop consistent modeling and validation approaches?
Climatology is a fast-evolving discipline, and knowledge about possible physical risks may change as scientists uncover new atmospheric dynamics. However, the largest source of uncertainty is future human behavior, which again depends on policies, new technologies, and societal preferences. As these uncertainties unfold, we must adapt the modeling to maintain consistency with the real world. Just as climate risk modeling is a tedious endeavor, so is the validation of climate risk models. Since historical relationships have limited explanatory power, validation should focus less on benchmarking historical events when testing models and rather use a wide range of possible scenarios as input to test the output of models.