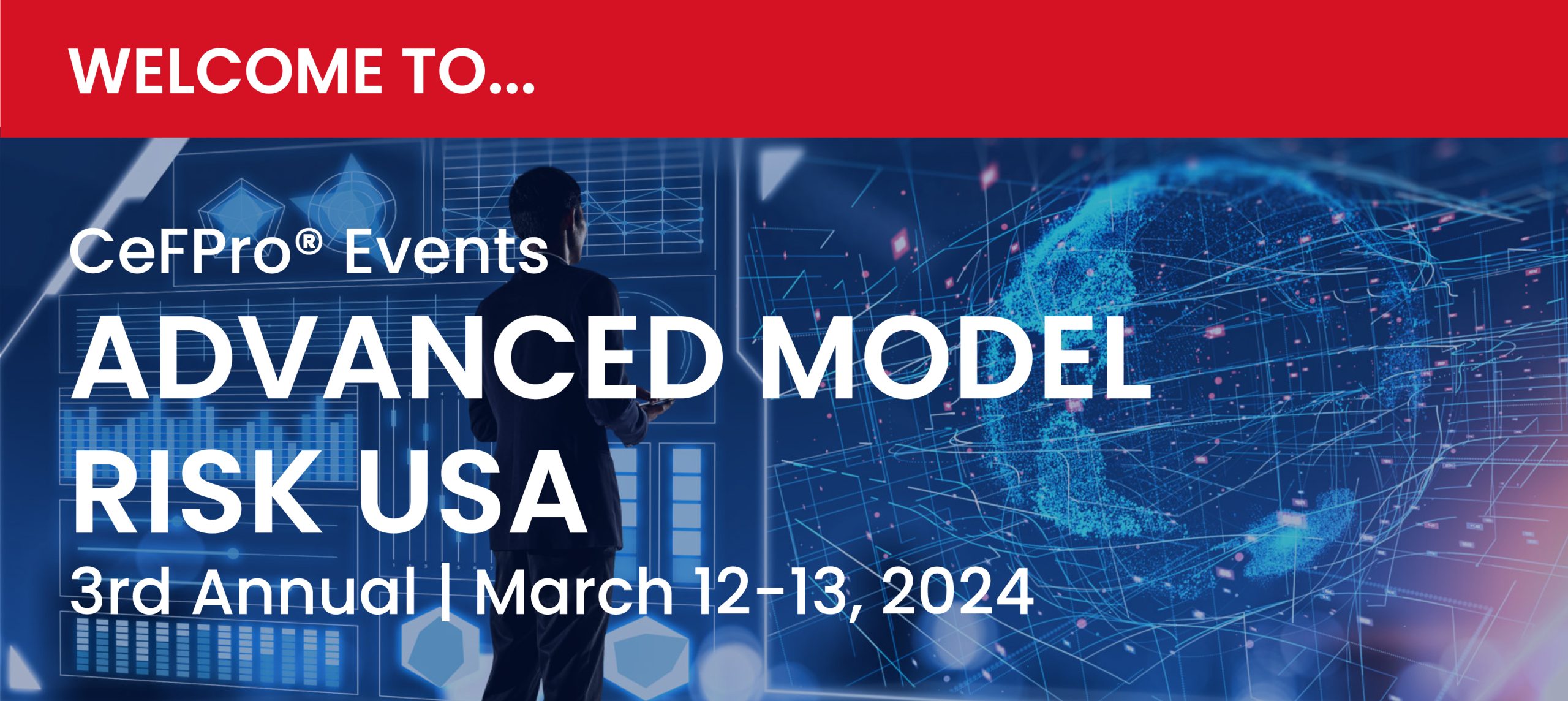
Advanced Model Risk Agenda
Day One | March 12
8:00-8:50
Registration and breakfast
8:50-9:00
Chairs opening remarks
Day 1 Moderator: Mehdi Esmail, Chief Product Officer, ValidMind
9:00-9:45
REGULATION – PANEL DISCUSSION
Enhancing model risk programs to manage divergence in regulatory expectations across jurisdictions
- Restructuring and leveraging existing resources to comply with existing regulatory expectations.
- Managing current market environment changes due to bank collapses.
- Navigating tighten regulatory expectations.
- Enhancing model risk programs to deal with AI regulations.
- Different standards of regulations between the US and Europe.
- Aligning standards of international banks with US regulations.
- Adjusting liquidity balance management models due to new regulation requirements.
- Technological expectations to comply with FRTB and Basel 4.
- Building capacity, governance, and infrastructure to meet requirements.
- Leveraging technology to streamline Model Governance and bridge gaps arising from diverse regulatory frameworks
- Adopting emerging considerations (AI/ML models, ESG, etc) for holistic model governance
Alberto Ramirez, FCA, MAAA, Partner – Risk Analytics, Solytics Partners
Oscar Zheng, Executive Director, Head of Model Validation, Natixis CIB Americas
Charlie Lu, Former Managing Director, Head of AI/ML Model Risk Management, Barclays
Nikolai Kukharkin, Head of Model Risk Management, MUFG
9:45-10:20
LARGE LANGUAGE MODELS
Adapting traditional model risk frameworks to align with large language models
- Testing and validating large language models
- Developing a forward-look approach to large language models
- Understanding the complexity of generative models for large language model validation
- Creating a platform to implement and develop large language models to mitigate risks
- Managing large language models beyond regulatory reporting
- Building use cases of large language models using NLP
- Controlling large language models whilst leveraging their functions
- Transparency and best practices for risk frameworks
Agus Sudjianto, EVP, Head of Corporate Model Risk, Wells Fargo
Tarun Joshi, Executive Director, NLP/ GEN AI Model Validation, Wells Fargo
10:20-10:50
Morning refreshment break and networking
10:50-11:25
VENDOR MODELS
A model builder’s perspective on AI/ML model risk in risk management
- Can hallucinations in risk management be tolerated?
- Can AI be useful in risk management?
- One size does not fit all – Considerations for capital models, trading models, monitoring models, risk management tools.
- Sharing some use cases.
- A vision for the future.
Hany Farag, Head of Modeling Methodology, CIBC
11:25-12:00
MACHINE LEARNING
Developing an effective AI and machine learning model risk management program
- Understanding machine learning lifecycle in banking
- Understanding key elements of machine learning model validation
- Testing modules for conceptual soundness
- Testing modules for outcome analysis
- Developing inherently interpretable benchmark models
- Developing the automated pipeline for streamlined validation
- Enabling automated validation and monitoring for dynamically updating models
Aijun Zhang, SVP, Head of Validation Engineering, Wells Fargo
12:00-12:35
From theory to practice: embedding AI risk management in the organizational fabric
- Understanding Risks from AI models and how they differ from other model risks
- An overview of the AI regulatory landscape
- How to quantify risks from models and Generative AI
- Establishing periodic model testing and evaluation protocols
- Risk mitigation strategies across the full model inventory
- Instituting robust governance structures to mitigate Organizational Risk
- Integrating end-to-end technological solutions and industry best practices to operationalize governance and build trustworthy AI systems
Anant Agrawal, Head of AI Risk Management, CIMCON Software
12:35-1:35
Lunch break and networking
1:35-2:10
BIAS AND EXPLAINABILITY
Assessing and monitoring AI, machine learning, and large language models to avoid bias and toxic results
- Safeguarding models to avoid reputational risk
- Balancing model performance, robustness, and fairness
- Going beyond traditional MRM functions to build models for explainability and bias testing
- Managing uncertainties of acquiring data to ensure models are not biased
- Transparency and explainability analysis within regulatory expectations
- Having a consistent approach to bias and explainability
- Managing stronger requirements for explainability of models in medium-to-long-term investing.
Roderick Powell, SVP, Head of Model Risk Management, Ameris Bank; NFR Leaders Advisory Board member, CeFPro
2:10-3:25
BLACK BOX MODELS – PANEL DISCUSSION
Understanding the methodology of black box models and increasing transparency
- Managing black box inputs and outputs
- Machine learning tools outside of vendor black box models
- Developing machine learning models and tools to validate internally
- Validating machine learning models outside of black box models
- Transparency with vendor black box models
- Approaching black box models from a different perspective to further understand
- Having a business approach
- Obtaining documentation and tools from vendors to understand how the model performs well
Seyhun Hepdogan, Director of Analytics, Fifth Third Bank; Fintech Leaders Advisory Board member, CeFPro
Moez Hababou, Head of Compliance, CCAR and Credit Models, BNP Paribas
Aleksey Leksanov, Managing Director, Head of Model Risk Management, Mizuho Bank
2:55-3:25
Afternoon refreshment break and networking
3:25-4:00
AI-powered SMB risk modeling
- Addressing challenges in SMB lending
- Role of AI in analyzing actionable insights from massive data sets
- Robust relationship analysis: enriching data with non-traditional niche sources
- Beyond conventional approaches: replacing traditional linear models, statistical distributions, and simple decision trees with modern machine learning methods
- End-to-end case management: Building a seamless SMB underwriting system
Sal Rehmetullah, Co-CEO and Co-Founder, Worth AI
Tom Aliff, SVP-Risk and Analytics, Equifax
4:00-4:35
AI GOVERNANCE
Operationalizing governance best practices of AI and machine learning models
- Integrating skill sets and disciplines
- Governing AI beyond a traditional model space
- Dealing with ethics, intellectual properties, reputational risks, and cyber security Psychology and linguistic experts to manage limitations of AI governance
- Governing AI beyond use case dependency
- Quantitative tools to measure and manage AI Modeling
- Meaningfully managing and governing AI/ml with model expansion
Rodanthy Tzani, Head of Model Risk Management, New York Life Insurance Company
4:35-5:10
MODEL INVENTORY
Managing complexities with the ongoing expansion of model risk scope and inventory
- Putting enhancements in place to accommodate AI
- Using 8 categories of Enterprise Risk Management for managing model governance and inventory
- Considering generative AI in model risk inventory
- Incorporating machine learning models into inventory
- ChatGPT and Chatbots
- Governance of new technologies and advanced models
- Developing replacements for model risk management in inventory
- Addressing the lack of inventory in risk management
Chris Smigielski, Director of Model Risk Management, Arvest Bank; NFR Leaders Advisory Board member, CeFPro
5:10-5:20
Chair’s closing remarks
5:20
End of day one
Day Two | March 13
8:00-8:50
Registration and breakfast
8:50-9:00
Chairs opening remarks
Day 2 Moderator: Chris Smigielski, Director of Model Risk Management, Arvest Bank; NFR Leaders Advisory Board member, CeFPro
9:00-9:40
GLOBAL VOLATILITY – PANEL DISCUSSION
Managing models with continued volatility and geopolitical challenges and the impact of change
- Using current data to cross reference climate impact on modeling portfolio
- Hurricanes, wildfire risks, flooding risks
- Climate change impacts on ability to provide loans
- Modelling physical and transition risk
- Defining climate risk and constituting where it falls within the model portfolio
- Managing the lack of data to foresee climate stress testing
- Understanding the impact of specific climate stress on the path of macroeconomic variable
- Standards for modeling climate risk
Alisa Rusanoff, Head of Credit, Crescendo Asset Management
Julia Litvinova, Managing Director, Head of Model Validation and Analytic, State Street
Deeptha Anand, Former, Head of Model Validation, Societe Generale
9:45-10:10
CLIMATE RISK –
Reviewing the impact of climate risk and incorporating within model risk management
- Using current data to cross reference climate impact on modeling portfolio
- Hurricanes, wildfire risks, flooding risks
- Climate change impacts on ability to provide loans
- Modelling physical and transition risk
- Defining climate risk and constituting where it falls within the model portfolio
- Managing the lack of data to foresee climate stress testing
- Understanding the impact of specific climate stress on the path of macroeconomic variable
- Standards for modeling climate risk
C.Robin Castelli, Author of Quantitative Methods for ESG Finance
10:10-10:40
Morning refreshment break and networking
10:40-11:10
Risks in AI
- an overview of high-profile recent model risk incidents
- focus areas for implementing AI risk management
- extending these methodologies to generative AI
- how technology can help
Jos Gheerardyn, Co-founder & CEO, Yields.io
11:10-11:40
AI GOVERNANCE
Mitigating overfitting risk in AI/ML models
- Model risk and overfitting
- Why AI/ML models have a tendency to overfit
- How to detect the overfit condition
- Mitigating techniques and methods
George Soulellis, Enterprise Model Risk Officer, Freddie Mac
11:40-12:20
MACROECONOMIC ENVIRONMENT – PANEL DISCUSSION
Understanding how models are incorporating macroeconomic effects and associated considerations
- Identifying key factors / macro drivers that models need to capture
- Adapting models for emerging risks using historical data and macro effects
- Adapting models for future macro scenarios outside of historical experience
- How do you manage the model limitations in the interim before you are able to recalibrate the models to capture the emerging risks?
- Challenges and enhancements of deposits modelling
- How do you make sure current models can calibrate successfully given current rates/economic environments, especially inverted yield curve?
- How can you make sure models will continue to calibrate successfully if and when Fed starts to reduce rates? What on-going monitoring mechanism would you put in place systematically?
Katherine Zhang, Managing Director, State Street
George Soulellis, Enterprise Model Risk Officer, Freddie Mac
Charles Shen, Managing Director, Head of Model Risk Management, Societe Generale
12:20-1:20
Lunch break and networking
1:20-1:50
QUANTIFYING MODEL RISK
Quantification of model risk and the aggregated model portfolio for end-to-end model risk management
- Assessing the overall risk of an interconnected model network
- Developing a quantitative measurement beyond risk ratings
- Practical and effective ways to quantify model risk
- Understanding the importance of an aggregated model portfolio to mitigate emerging risks
- Intersection and connection point between models
- Verifying and monitoring the data between model
Manoj Singh, Managing Director, Model Risk Officer, Bank of America
1:50-2:20
DATA
Managing the evolution of data requirements as model requirements expand
- Incorporating Covid data into modeling
- Controlling covid data
- Updating models using credit loss forecasting models
- Should Covid data be included in these?
- Addressing unpredictable Covid data in the models
- Ensuring models predict credit loss appropriately
- Addressing credit loss due to unprecedented scenarios
- Pandemics
Imir Arifi, Head of AI Model Development, UBS
2:20-2:50
FRAUD & FINANCIAL CRIME
Managing the increase in fraud and financial crime tools in model risk management inventory
- Gathering data to govern and review financial crime tools
- Fitting in tools into the traditional definition of models
- Clearly defining financial crime models with regulatory expectations
- Getting appropriate results from quantitative modeling tools
- Evaluating conceptual soundness to ensure the model is fit for use
- Including new dimensions and updating models to capture fraud
- Capturing potential fraudulent events using fraud detection modeling
- Building systems to flag potential threats
Ankur Goel, SVP, Head of Consumer and Fraud Modeling, PNC
2:50-3:20
Afternoon break and networking
3:20-3:50
PERFORMANCE MONITORING
Strengthening model risk management through ongoing performance monitoring
- Regulatory expectations of ongoing performance monitoring of model risk
- Tracking and validating model performance
- Addressing the thresholds of ongoing model performance monitoring
- Setting tolerance levels on performance
- Incorporating automation in ongoing performance monitoring
- Identifying risks between performance reviews
Arthur Robb, Managing Director, Head of Model Risk Management, TIAA
3:50-4:20
CREDIT RISK
Incorporating emerging credit risks into model risk management frameworks and measuring exposure
- Measuring model exposure to a high inflation environment
- Limitations of models and anticipation of risks
- Adapting concurrent views of model risk to mitigate counterparty credit risk
- Incorporating modeling metrics into daily risk management
- Incorporating overlays into models for credit risk management
- Balance sheet and trading book positioning for decision-making
- Alignment for business changes in the horizon on credit risk
- Developing credit risk modeling strategies
Daniel Saunders, Head of Model Risk, USAA
4:20
Chair’s closing remarks
4:30
End of Congress
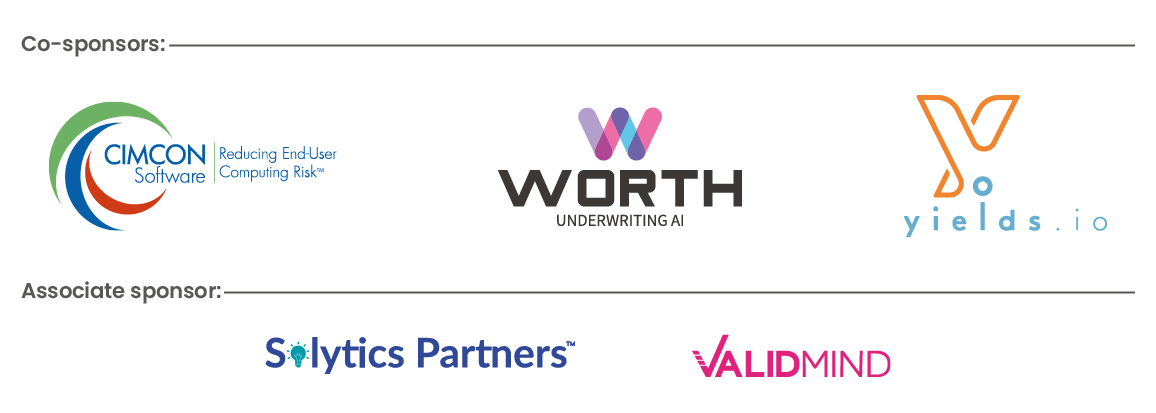