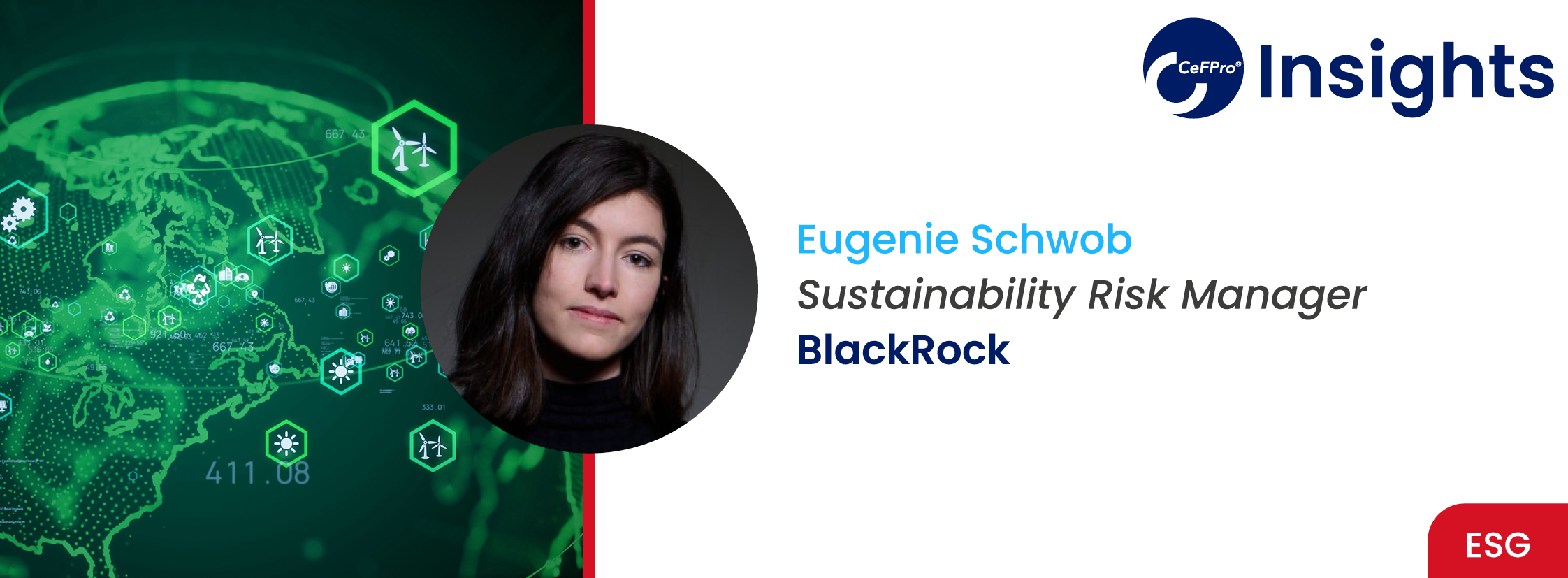
Reviewing the evolution of climate data
Eugenie Schwob, Sustainability Risk Manager, BlackRock
Below is an insight into what can be expected from Eugenie’s session at Climate Risk USA 2023.
Disclaimer: The views and opinions expressed in this article are those of the thought leader as an individual and are not attributed to CeFPro or any particular organization.
How has climate data evolved over recent years?
Climate data availability and coverage have increased in recent years, especially for climate transition data.
For climate transition data, one of the most common metrics is historical carbon emissions (whether absolute, intensity by sales, or intensity by enterprise value). While in 2008, only 40% of MSCI World Index’s companies by market value had reported absolute scope 1 & 2 carbon emissions, in 2020, that proportion has more than doubled, with almost 90% reported emissions and the rest being mostly estimated.
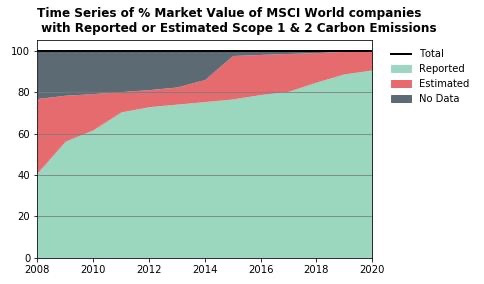
While carbon emissions are a critical metric to measure for a given company and can be a great starting point for portfolio analysis, looking at this metric on its own has limitations. Indeed, transition risk is not only about past emissions but also how companies are transitioning, whether through their operational journey (going from carbon-intensive to more efficient operations) and/or through their business model journey (going from making high carbon-intensive products and services to low carbon-intensive ones). Each lens of analysis requires different types of data metrics:
- On the operational side, looking at targets for emissions reductions the company has set, whether these are credible, and whether the company is on track to meet them is critical. These can also inform modeled projected emissions or temperature alignment assessment.
- On the business model side, looking at revenues from mitigation activities or CAPEX and R&D spending by the company helps understand how or if the company is transforming its business model.
Finally, more sophisticated transition risk analytics tend to require the use of climate scenarios or at least a better understanding of how a company is sensitive to transition macro factors. These are still evolving data points.
For climate physical data, the data landscape is still more sparse. Geolocation-based data combined with scenario-based hazard climate data helps assess financial impact. However, more work is being done on event analysis and stress testing. A key question for climate physical data is around time horizon and how to model best the impacts that both chronic and acute physical events can have on shorter-term investments.
Why is it important for asset managers to integrate climate data into their strategy?
Asset managers have a fiduciary duty to their clients to pursue the best investment outcomes within the mandates they are given. By acting on behalf of institutional and individual investors and transacting for investor clients, not for themselves, investment managers must act in the best long-term interests of their beneficiaries. It means a requirement upon all trustees to consider current and evolving risks when making investment decisions. It is, therefore, important to consider financially material climate data or information with the objective of enhancing the risk-adjusted returns of clients’ portfolios. This applies regardless of whether a fund or strategy has a sustainable or ESG-specific objective.
How can asset managers ensure consistent structured and unstructured data is obtained across the organization?
Centralized data and data access is key. Different teams across the organization may have different expertise – some research analysts may have a good understanding of the company’s strategy under a low-carbon transition scenario, while another investor may be more familiar with the firm’s current balance sheet and valuation. To bring it together and allow such expertise to exchange views can be more easily done if everyone in the organization looks at the same underlying data or starting point.
As an example, companies’ commitments or emissions reduction targets are not as straightforward a data point to get access to. While the raw data can be sourced from public reports, such as TCFD, CDP disclosures, SBTi, or the company’s own sustainability reports, reconciling these different sources into a piece of unique information can be challenging. Indeed, it is not always clear what is being covered in terms of scope and time horizon, nor which subsidiaries are included in the commitment. Research analysts who cover the specific company may have the best understanding of the exact details of the target and whether it would apply to a certain subsidiary or not. By looking at centralized data, they can raise issues and, therefore, ensure that the data otherwise systematically collected is valid.
Why should asset managers conduct due diligence on third party providers? How does this impact their climate data?
Third party data vendors for ESG data play an important role in the financial industry. These data vendors may provide thousands of data points or Key Performance Indicators (“KPI”). From these KPIs, a subset is often used for key firmwide use cases, such as regulatory reporting, client reporting, or broad portfolio objectives.
Given that scale impact, it is critical that this smaller subset is being used appropriately and transparently. This can be done through better due diligence of the data points provided by the third-party providers, as well as ensuring their appropriateness for the specific use case. To achieve this, a combination of qualitative and quantitative analysis is needed to better understand the biases and limitations of the data and to document these explicitly before providing approval for usage on behalf of the firm. Key considerations include coverage, quality of the underlying data being used, transparency in the methodology, understanding of the assumptions, if any, correlation with other data points, and stability of the output.
Especially for climate data, which is still nascent, one may rather have a smaller dataset of reliable data points (companies’ targets, green bonds, revenues split) rather than leverage overly simplistic proxy techniques that cover a bigger universe of securities.