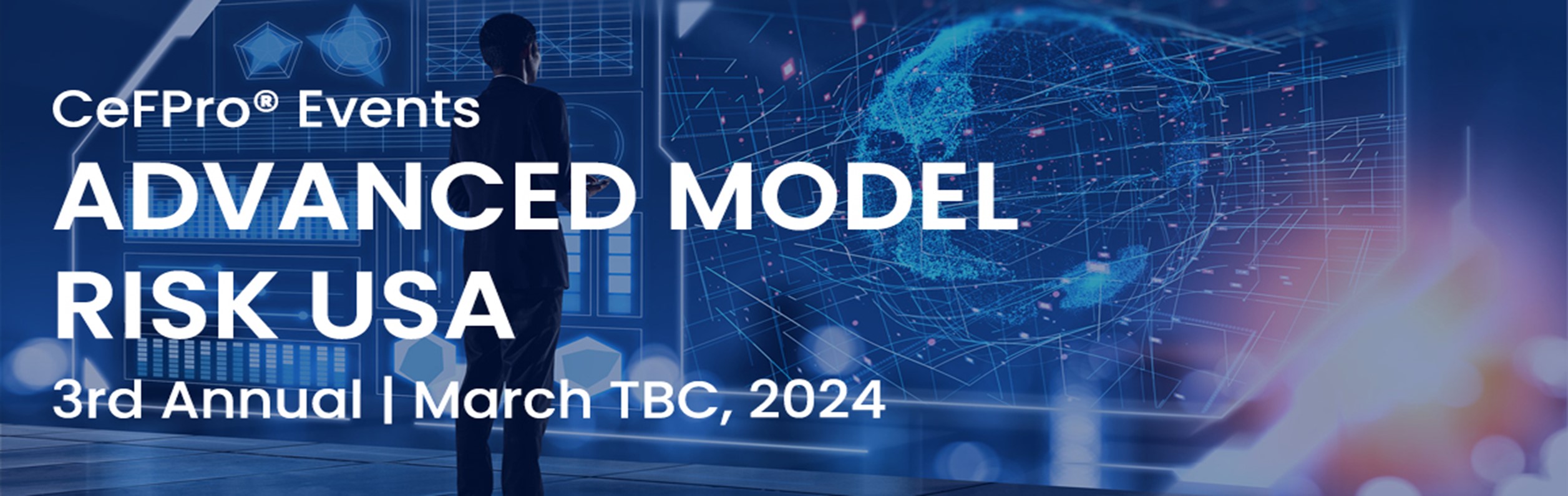
Agenda
8:00 – 8:50
Registration and breakfast
8:50 – 9:00
Chair’s opening remarks
9:00 – 9:45
REGULATION – PANEL DISCUSSION
Enhancing model risk programs to manage divergence in regulatory expectations across jurisdictions
View Session Details
- Restructuring and leveraging existing resources to comply with existing regulatory expectations
- Managing current market environment changes due to bank collapses
- Navigating tighten regulatory expectations
- Enhancing model risk programs to deal with AI regulations
- Different standards of regulations between the US and Europe
- Aligning standards of international banks with US regulations
- Adjusting liquidity balance management models due to new regulation requirements
- Technological expectations to comply with FRTB and Basel 4
- Building capacity, governance, and infrastructure to meet requirements
![]() |
Xiangyin (Jane) Zheng, Audit Director, BNY Mellon |
![]() |
Oscar Zheng, Executive Director, Head of Model Validation, Natixis CIB Americas |
![]() |
Wei Zhu, Managing Director, Citi |
9:45 – 10:20
COMPLIANCE
Defining a model to ensure compliance with regulatory expectations
View Session Details
- Defining a standard for what constitutes a model
- Classifying a clear definition of a modeling tool or solution
- Approaches to dictating if a tool or solution is categorized as a model
- MRM teams vs. SR117’s definitions of a model
- Constituting a model and if it needs to be validated outside of SR117’s guidance
- Managing the challenges and scope of validation requirements
- Managing model risk as a risk and a compliance function
- Looking at model risk beyond a one-size-fits-all all approach
![]() |
Janet Shand, SVP & Director, Model Risk Management, NYCB |
10:20-10:50
Morning refreshment break and networking
10:50-11:25
GENERATIVE AI
Understanding advances of generative AI and incorporating into model risk management to mitigate risk
View Session Details
- Looking at AI beyond a model risk management framework
- Increasing training to understand generative AI to fill gaps
- Risk management framework vs. model risk management framework
- Identifying methods to put governance into action for generative AI
- Addressing the multiple tools and business outcomes of generative AI
- Utilizing generative AI to drive efficiency and testing technical aspects
- Risk rating multiple generative AI type models
- Including privacy and legal teams
- Testing the accuracy of AI and generative AI
11:25-12:00
MACHINE LEARNING
Developing an effective AI and machine learning model risk management program
View Session Details
- Increasing number of machine learning models and tools
- Understanding different algorithms and methodologies
- Defining who is responsible for machine learning model risk management
- Data governance in developing machine models
- Learning landscape and following regulatory changes
- Machine learning beyond traditional model risk testing
- Breaking down complexity in methodology, transparency, and technology
- Understanding AI/ML methodologies and non-transparent principles
- Approaching and identifying key risk factors to ensure AI/ML models are fit for purpose
![]() |
Aijun Zhang, SVP Machine Learning & Validation Engineering, Wells Fargo |
12:00-12:35
LARGE LANGUAGE MODELS
Adapting traditional model risk frameworks to align with large language models
View Session Details
- Testing and validating large language models
- Developing a forward-look approach to large language models
- Understanding the complexity of generative models for large language model validation
- Creating a platform to implement and develop large language models to mitigate risks
- Managing large language models beyond regulatory reporting
- Building use cases of large language models using NLP
- Controlling large language models whilst leveraging their functions
- Transparency and best practices for risk frameworks
![]() |
Agus Sudjianto, EVP, Head of Corporate Model Risk, Wells Fargo |
12:35-1:35
Lunch break and networking
1:35-2:10
BIAS AND EXPLAINABILITY
Assessing and monitoring AI, machine learning, and large language models to avoid bias and toxic results
View Session Details
- Safeguarding models to avoid reputational risk
- Balancing model performance, robustness, and fairness
- Going beyond traditional MRM functions to build models for explainability and bias testing
- Managing uncertainties of acquiring data to ensure models are not biased
- Transparency and explainability analysis within regulatory expectations
- Having a consistent approach to bias and explainability
- Managing stronger requirements for explainability of models in medium-to-long-term investing.
![]() |
Roderick Powell, SVP, Head of Model Risk Management, Ameris Bank |
2:10-3:25
BLACK BOX MODELS – PANEL DISCUSSION
Understanding the methodology of black box models and increasing transparency
View Session Details
- Managing black box inputs and outputs
- Machine learning tools outside of vendor black box models
- Developing machine learning models and tools to validate internally
- Validating machine learning models outside of black box models
- Transparency with vendor black box models
- Approaching black box models from a different perspective to further understand
- Having a business approach
- Obtaining documentation and tools from vendors to understand how the model performs well
![]() |
Seyhun Hepdogan, Director of Analytics,Fifth Third Bank |
![]() |
Moez Hababou, Head of Compliance, CCAR and Credit Models, BNP Paribas |
![]() |
Stephen Hsu, SVP, Head of Model Risk Management, Pacific Western Bank |
![]() |
Janet Shand, SVP & Director, Model Risk Management, NYCB |
2:55-3:25
Afternoon refreshment break and networking
3:25-4:00
AI GOVERNANCE
Operationalizing governance best practices of AI and machine learning models
View Session Details
- Integrating skill sets and disciplines
- Governing AI beyond a traditional model space
- Dealing with ethics, intellectual properties, reputational risks, and cyber security
- Psychology and linguistic experts to manage limitations of AI governance
- Governing AI beyond use case dependency
- Quantitative tools to measure and manage AI Modeling
- Meaningfully managing and governing AI/ml with model expansion
![]() |
Rodanthy Tzani, VP, Head of Model Risk Management,New York Life insurance Company |
4:00-4:35
MODEL INVENTORY
Managing complexities with the ongoing expansion of model risk scope and inventory
View Session Details
- Putting enhancements in place to accommodate AI
- Using 8 categories of Enterprise Risk Management for managing model governance and inventory
- Considering generative AI in model risk inventory
- Incorporating machine learning models into inventory
- ChatGPT and Chatbots
- Governance of new technologies and advanced models
- Developing replacements for model risk management in inventory
- Addressing the lack of inventory in risk management
![]() |
Chris Smigielski, Director of Model Risk Management, Arvest Bank |
4:35-4:45
Chair’s closing remarks
4:45
End of day one and drinks reception
8:00 – 8:50
Registration and breakfast
8:50 – 9:00
Chair’s opening remarks
9:00 – 9:45
GLOBAL VOLATILITY – PANEL DISCUSSION
Managing models with continued volatility and geopolitical challenges and the impact of change
View Session Details
- Addressing threat rates to models
- Impact of current world changes and changes in structures and capabilities
- Managing models based on geopolitical challenges
- S. election cycles, wars, European Union, stagflation in China
- Addressing the energy crisis and the impact on model risk
- Impact of high inflation and rising rates on models
- Model function changes in high rate vs. low rate risk environments
- Impact of investment strategies in modeling portfolios
- Short-term investment vs. medium to long-term investing
- Capturing data to manage the behavior of market regimes
![]() |
Alisa Rusanoff, Head of Credit, Crescendo Asset Management |
![]() |
Julia Litvinova, Managing Director, Head of Model Validation and Analytic, State Street |
![]() |
Deeptha Anand, Head of Model Validation, Societe Generale |
9:45-10:20
CLIMATE RISK –
Reviewing the impact of climate risk and incorporating within model risk management
![]() |
C.Robin Castelli, Head of Transition Risk Model Development, Citi |
10:20-10:50
Morning refreshment break and networking
10:50-11:25
CREDIT RISK
Incorporating emerging credit risks into model risk management frameworks and measuring exposure
View Session Details
- Measuring model exposure to a high inflation environment
- Limitations of models and anticipation of risks
- Adapting concurrent views of model risk to mitigate counterparty credit risk
- Incorporating modeling metrics into daily risk management
- Incorporating overlays into models for credit risk management
- Balance sheet and trading book positioning for decision-making
- Alignment for business changes in the horizon on credit risk
- Developing credit risk modeling strategies
11:25-12:10
MACROECONOMIC ENVIRONMENT – PANEL DISCUSSION
Understanding how models are measuring interest rate exposure and recalibrating based on market changes
View Session Details
- Identifying key factors of interest rate risk that models need to capture
- Adapting models for emerging risk using historical data
- Adapting model risk processes to identify weaknesses in the framework
- Addressing limitations imposed by regulators and monetary
- Measuring risks accurately and having a sufficient view of measuring interest rate risk
- Incorporating important and significant elements
- ALM – data science problem vs. accounting problem
![]() |
Katherine Zhang, Managing Director, State Street |
![]() |
Charlie Lu, Former Managing Director, Head of AI/ML Model Risk Management, Barclays |
12:10-1:10
Lunch break and networking
1:10-1:45
QUANTIFYING MODEL RISK
Quantification of model risk and the aggregated model portfolio for end-to-end model risk management
View Session Details
- Assessing the overall risk of an interconnected model network
- Developing a quantitative measurement beyond risk ratings
- Practical and effective ways to quantify model risk
- Understanding the importance of an aggregated model portfolio to mitigate emerging risks
- Intersection and connection point between models
- Verifying and monitoring the data between model
![]() |
Manoj Singh, Managing Director, Model Risk Officer, Bank of America |
1:45-2:20
DATA
Managing the evolution of data requirements as model requirements expand
View Session Details
- Incorporating Covid data into modeling
- Controlling covid data
- Updating models using credit loss forecasting models
- Should Covid data be included in these?
- Addressing unpredictable Covid data in the models
- Ensuring models predict credit loss appropriately
- Addressing credit loss due to unprecedented scenarios
- Pandemics
2:20-2:55
VENDOR MODELS
Leveraging vendor models and building out effective oversight capabilities to align with internal governance and controls
View Session Details
- Understanding the risk and controls with third parties and model risk management
- Building relationships with third parties for model risk management
- Having transparency with third parties
- Reviewing vendor documentations
- Developing internal models to mitigate third-party risks
- Capabilities and resources
- Aligning with vendors to mitigate impacts and risk
- Performance measures of vendor models
- Model validation support to ensure new systems meets all requirement
- Managing constant changes from the compliance side
![]() |
Hany Farag, Head of Modelling Methodology, CIBC |
2:55-3:25
Afternoon refreshment break and networking
3:25-4:00
PERFORMANCE MONITORING
Strengthening model risk management through ongoing performance monitoring
View Session Details
- Regulatory expectations of ongoing performance monitoring of model risk
- Tracking and validating model performance
- Addressing the thresholds of ongoing model performance monitoring
- Setting tolerance levels on performance
- Incorporating automation in ongoing performance monitoring
- Identifying risks between performance reviews
![]() |
Arthur Robb, Managing Director, Head of Model Risk Management, TIAA-CREF |
4:00-4:35
FRAUD & FINANCIAL CRIME
Managing the increase in fraud and financial crime tools in model risk management inventory
View Session Details
- Gathering data to govern and review financial crime tools
- Fitting in tools into the traditional definition of models
- Clearly defining financial crime models with regulatory expectations
- Getting appropriate results from quantitative modeling tools
- Evaluating conceptual soundness to ensure the model is fit for use
- Including new dimensions and updating models to capture fraud
- Capturing potential fraudulent events using fraud detection modeling
- Building systems to flag potential threats
![]() |
Ankur Goel, SVP, Head of Consumer and Fraud Modelling, PNC |
4:35-4:45
Chair’s closing remarks
4:45
End of Congress